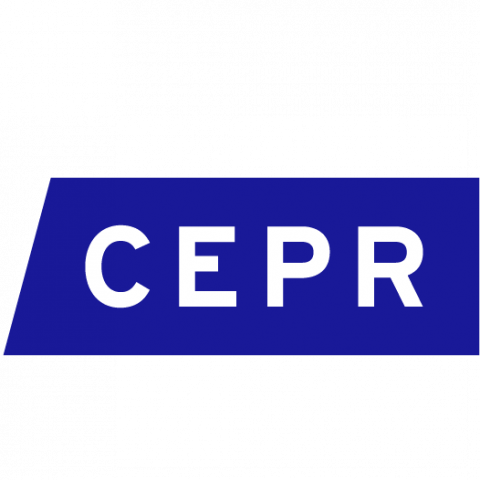
This section presents an overview of different approaches to measuring climate change adaptation, as well as their advantages and disadvantages. Two things are worth noting before we undertake a detailed description of these methods. First, some of the studies cited used data from developed countries, but they are relevant to this review because of their methodological contribution, and later in the review we highlight where these methods might diverge when using data on developing countries. Second, “climate” and “weather” by definition include a large number of phenomena that are relevant for economic outcomes in developing countries, including (but not limited to) temperature, rainfall patterns, and a multitude of extreme events such as hurricanes and floods. Most studies consider a subset of these (e.g. either temperature and rainfall or hurricanes) when estimating impacts.
Cross-sectional studies
Climate change manifests through rising temperatures, unpredictable rainfall patterns, and increasingly frequent natural disasters. The earliest studies estimating the economic impacts of climate change relied on agronomy models of the impact of heat exposure on plants to quantify how higher temperatures would impact agricultural yields, which have over time developed into models that allow for several important agronomic factors (Rosenzweig et al. 2014).
To understand how agricultural profits (rather than yields) would respond to climate change, Mendelsohn et al. (1994) used cross-sectional data on agricultural land values in the US and regressed them on 30-year average temperature and rainfall variables (using linear and quadratic terms for each of these variables to allow for nonlinear effects of climate), as well as control variables for soil, market access, and related confounders. The rationale behind using land prices was that these variables should capitalise the long-term value of climate in a location (which could be negative), and the advantage of using long-term weather averages was that it allowed for adaptation and estimated the effects of climate change net of adaptation. Using the estimated marginal values of temperature and precipitation, they predict land values in each county using the current climate, and then using the future climate, allowing them to compute the impacts of climate change. The results from this study predicted small impacts of climate change on US agriculture.
Follow-up studies focused on other countries, including developing countries, and additionally directly estimated the returns to adaptation, such as irrigation (Seo et al. 2005, Kurukulasuriya et al. 2011), finding large negative impacts of climate change on agricultural profits in developing countries. However, a drawback of these studies is that the primary right-hand side variables are cross-sectional (i.e. 30-year average temperature and precipitation), meaning omitted variable bias may be a concern when interpreting these estimates – climate may be correlated with infrastructure quality or other variables which are omitted from the regression specification. If so, the estimated impact of climate may be biased.
Identification via weather shocks
Given the identification concerns with cross-sectional approaches, follow-up work relied on using weather shocks, rather than long-term climate, when estimating the impacts of climate change. These papers (Deschênes and Greenstone 2007, 2012, Fisher et al. 2012, Graff et al. 2014) used panel data on agricultural profits and weather variables (temperature and precipitation) to estimate how weather shocks, usually measured as annual or seasonal deviations from long-term climate, impacted agricultural profits in the US. Using these weather variables, Deschênes and Greenstone (2012) find predicted losses of about $4 billion for US agriculture by the end of the century when extrapolating to future predicted climate scenarios (they use values of agricultural profits predicted using the impacts of weather shocks, and the projected climate for each county). Similar to the cross-sectional approaches, these studies usually extrapolate the estimated marginal effects of climate or weather to a future climate scenario to compute how climate change would affect the outcome of interest in the future.
A significant advantage of this approach is that the use of panel data methods mitigates omitted variable bias. For this reason, this approach has been subsequently influential and currently is the most used approach in estimating the impacts of weather shocks and climate. A potential disadvantage is the uncertainty regarding extrapolating the impact of say, one hotter year by two degrees Celsius, to a world that is permanently warmer by two degrees. The impact of a weather shock may be an overestimate relative to a changed climate if some adaptations may be available in the longer term that are not available in the short run (e.g. switching crop varieties, or innovation of more heat-resistant varieties in the even longer term). In contrast, if short-run adaptations are possible that are infeasible in the longer term, e.g. excessive pumping of groundwater to irrigate and mitigate the impact of a hotter year, weather shocks may provide an underestimate of climate change (see Lemoine 2018 for a detailed treatment of these issues).
More recently, work in developing countries has estimated the impacts of weather shocks on a diverse set of economic outcomes, ranging from agricultural profits, worker and firm productivity, and economic growth. Several of these studies also consider adaptation, which we discuss in the next section. Dell et al. (2012) show that higher temperatures substantially reduce economic growth in poor countries, adversely impacting both agricultural and industrial output. Guiteras (2009) finds that weather shocks reduce crop yields by about 5% to 9% in India, with longer-term damages estimated to be 25% of crop yields in the absence of adaptation. Somanathan et al. (2021) use plant-level Annual Survey of Industries (ASI) data on manufacturing firms in India and find reductions of 2% of annual output per one degree Celsius of hotter temperatures. Zheng et al. (2018) estimate an inverted U-shaped relationship between temperature and TFP in China, with both labour- and capital-intensive firms exhibiting sensitivity to high temperatures. Other work has focused on how weather shocks impact labour markets, such as the intersectoral allocation of labour (Colmer 2021, Santangelo 2019). The negative impacts of weather are present not only when considering annual level shocks, but also daily variation. Using data for blue-collar (garment factory) workers in India, Adhvaryu et al. (2020) find that daily temperatures exceeding about 85 degrees Fahrenheit substantially lower production-line level productivity that day, an effect that is not driven by selection into the type of workers that attend work on hotter days.
In addition, recent work has found the effects of hotter years on human capital formation in developing countries. Garg et al. (2020) show that high temperatures reduce math and reading test scores among school-age children, an effect that is higher during agricultural growing seasons, when hotter temperatures also reduce crop yields. Even beyond contemporaneous effects of heat on education, Fishman et al. (2019) find that in-utero exposure to an additional degree celsius of temperature has long-term effects on education and earning. Shah and Steinberg (2017), in contrast, find that contemporaneous positive rainfall shocks increase child labour for children aged five and higher, and lower human capital investment, as they increase the opportunity cost of spending time in school for children.
Related work has also examined the impacts of weather shocks on infant mortality and mortality more broadly. For instance, Burgess et al. (2017) find that hotter years in India increase mortality within a year of their occurrence, an effect that is present in rural households. Furthermore, hotter and drier years also reduce agricultural output as well as wages. Banerjee and Maharaj (2020) find negative impacts of hotter years on infant mortality in India and also test whether workfare programmes and community health programmes can mitigate this relationship (described in more detail in the next section). Geruso and Spears (2018) use Demographic and Health Survey (DHS) data from 53 developing countries, and similarly find negative impacts of hotter years on infant mortality. In terms of magnitude, their estimated effect sizes are large, comparable to similar estimates in the US, prior to air conditioning adoption, between 1930 and 1959. Furthermore, some papers test whether exposure to early-life weather shocks negatively impacts later-life outcomes. Maccini and Yang (2009) find that exposure to higher early-life rainfall in the year of birth leads to improved health, schooling, and socioeconomic status for women in the Philippines, with no effect of higher rainfall in-utero. Shah and Steinberg (2017) find that positive rainfall shocks in-utero and early in life increase the likelihood of a child being in school in India.
Other important economic outcomes in developing countries impacted by warming and rainfall shocks include crime as well as civil conflict (see Burke et al. (2015) for a comprehensive review). Burke et al. (2015) evaluate data from 60 studies using 45 different types of conflict data and find that higher temperatures and lower rainfall increase conflict across the developing world. Blakeslee and Fishman (2018) use data on crime and weather in India between 1971 and 2000 and find that hotter years and drought years increase all types of crime. Such shocks can also increase gender-based violence such as dowry deaths in India (Sekhri and Storeygard 2014) and witch killings in Tanzania (Miguel 2005). While lower income or resources leading to conflict is one likely mechanism that is often found to be important in explaining the effects of these shocks (McGuirk and Nunn 2021), it is often not sufficient to explain the entire impact, indicating that the complete set of mechanisms via which weather shocks impact conflict and crime are not yet fully understood.
In addition to estimating the impacts of weather on final economic outcomes such as productivity, profits, and crop yields, this literature has also looked at how different natural disasters impact economic outcomes. Developing countries have among the highest occurrence of natural disasters, but more importantly, have the highest number of people affected by them – see Figure 3 reproduced using EMDAT data (Guha-Sapir et al. 2023). Hsiang and Jina (2014) find that cyclones reduce economic growth for both rich and poor countries, with persistently negative impacts on economic growth. Using panel data on Indian firms, Pelli et al. (2023) find that hurricanes impact firms’ exit rates and sales, and these effects are larger for less productive firms. They also find evidence that after the hurricane, firms respond by abandoning production in industries with lower comparative advantage. Elliot et al. (2015) use nightlights data to estimate how typhoons impact short-run local economic activity in China - they find that a typhoon estimated to destroy 50% of the property reduces local economic activity by 20% in the same year, with total net economic losses from typhoons estimated to be about $28 billion between 1992-2010.
Baez and Santos (2007) find that children in regions that were affected by Hurricane Mitch in Nicaragua had worse health outcomes as well as a lower probability of seeking healthcare conditional on being ill. Caruso (2017) combines census data for Latin America and the Caribbean with data on natural disasters and finds that disasters negatively impact educational attainment for affected children, with impacts varying by the age of exposure to disaster, the type of natural disaster, as well as the place and time of exposure. The results raise important questions about the ability to extrapolate impacts from one country to another and show the persistent negative impacts disasters can have on impacted populations. The paper also finds a U-shaped relationship between GDP per capita and impacts on educational attainment.
Figure 3: Global occurrences of natural disasters and impacted populations (EMDAT Database)
Panel A: Global occurrences of natural disasters by country between 1980 - 2023
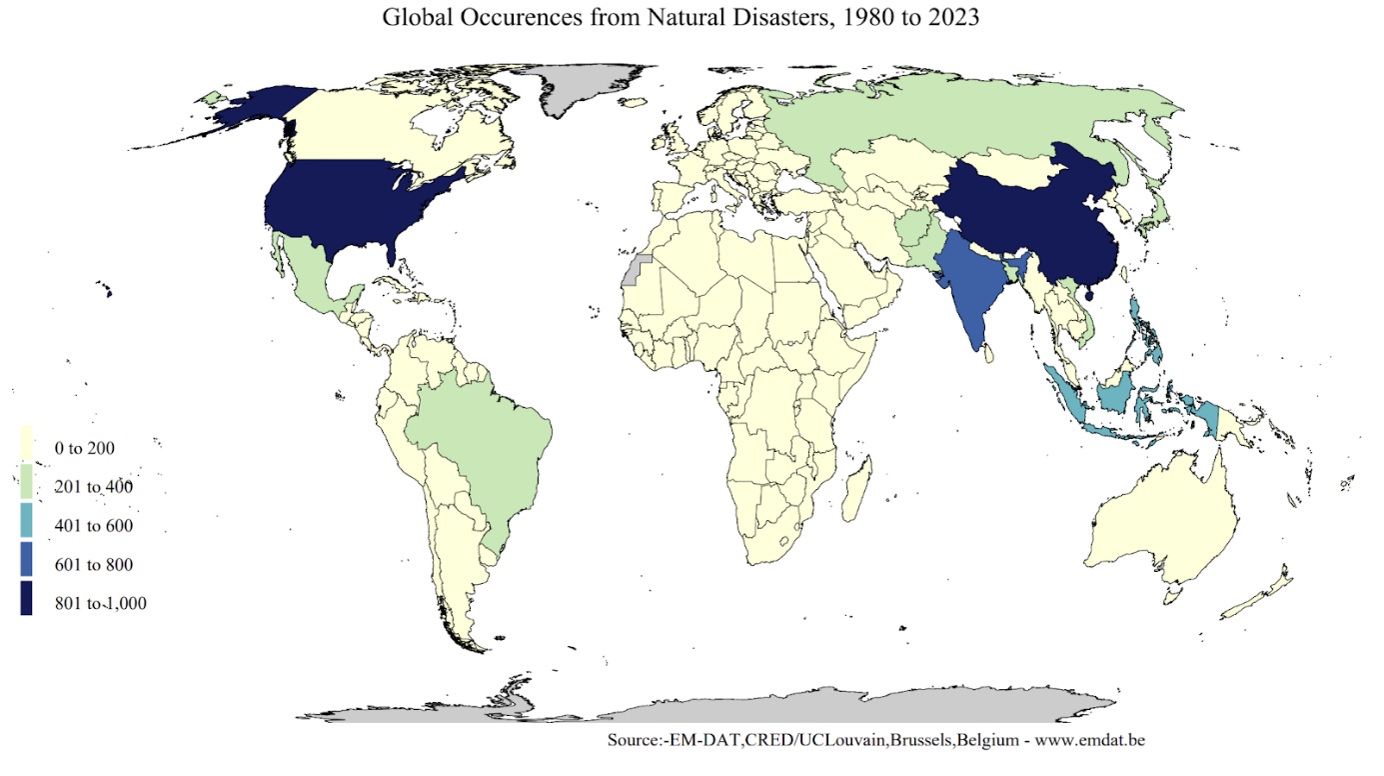
Panel B: Total population affected by natural disasters by country between 1980 - 2023
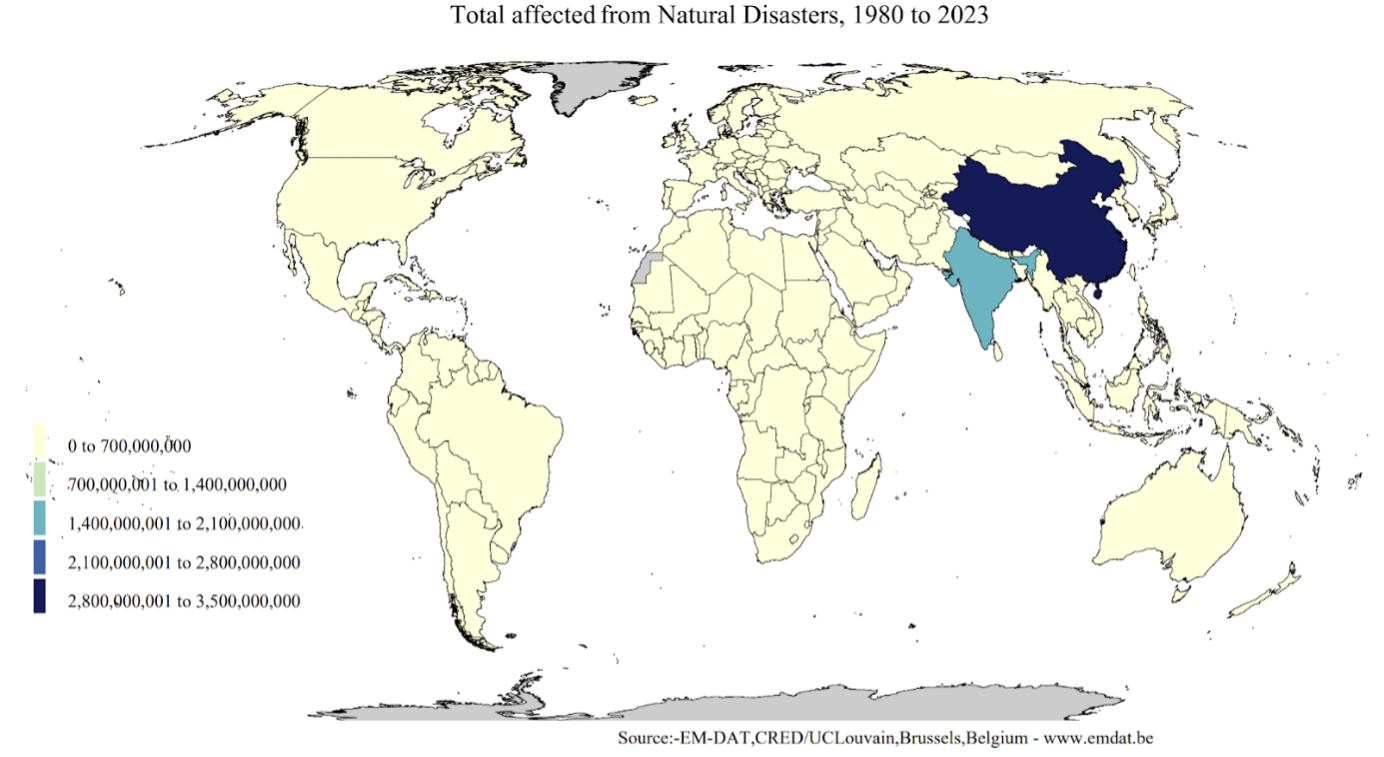
Recent methodological developments
This section reviews some recent methodological innovations in estimating climate impacts and adaptation. The first such approach is using long-run (for instance, decadal) changes in temperature and precipitation, which avoid some concerns regarding the lack of adaptation when estimating the impact of short-run weather shocks. Burke and Emerick (2016) estimate the impact of decadal changes in temperature and precipitation on maize and soy yields in the US and find large negative impacts in counties with greater decadal warming. They also find limited to no adaptation, indicating that losses from climate change are likely to be large for these crops. Liu et al. (2023) use long differences to test how decadal changes in temperature impact the sectoral allocation of labour and urbanisation in India. They find that a one degree Celsius increase in temperature in an average district in India increases the share of the labour force in agriculture by 17% and decreases the share of the labour force in non-agriculture by 8%, with no impact on urbanisation (although Henderson et al. (2017) find heterogeneity in the effects of climate shocks on urbanisation with positive effects when the cities are manufacturing centers). This approach incorporates the role of adaptation partially by estimating the impact of longer-run weather patterns but may have the drawback of limited support over which impacts can be estimated.
The second approach is to use the differences in the impacts between trends in warming and short-run temperature shocks to quantify the extent of adaptation. This is the approach taken by Bento et al. (2020), who use it to quantify the impact of climate change on atmospheric ozone concentration to detect adaptation (an absence of adaptation would imply similar ozone concentrations for given temperature changes). The third approach is to use machine learning to identify which weather variables are quantitatively important in determining economic outcomes such as crop yields, rather than relying on particular functional forms or weather variables. For instance, Hultgren et al. (2022) use machine learning approaches to estimate how weather shocks impact crop yields for six crops globally over time, finding considerable negative effects – they find that a one degree rise in global mean temperature reduces crop yields by about 4.5%, with limited adaptation. This approach has the distinct advantage that it does not rely on the researcher to specify the correct functional form of the relationship between weather and economic outcomes.
Expectations
A different but related set of papers seek to understand how households’ and firms’ expectations regarding the weather and the climate impact their adaptation decisions. For instance, Shrader (2017) uses forecasts to understand their role in adaptation to El Nino/Southern Oscillation (ENSO) variation for albacore tuna harvesters in the North Pacific US. He finds that the benefits of forecasts in this setting are large, with the forecast allowing farmers to adapt to ENSO changes. Some caution is in order when extending these results to developing countries. In places where forecasts are trustworthy, they can facilitate adaptation to short-run weather. However, weather phenomena which are important for economic outcomes in developing countries may be more difficult to forecast – for instance, Rosenzweig and Udry (2013) show that the correlation between long-range monsoon forecasts and realised rainfall is very small in India, which implies that most farmers would rationally ignore this information. To capture the potential uncertainty caused by climate change in farmer decisions, Kala (2017) develops an empirical framework to test how farmers respond to the Knightian uncertainty possibly inherent in learning about a changing climate, i.e. in the short and medium term, farmers may not even know whether the climate has changed. The paper finds that farmers’ beliefs about monsoon onset are consistent with such learning behaviour, but only in villages that have experienced greater changes to the rainfall distribution in recent decades.
Other work has also looked at how expectations regarding climate change – namely climate change forecasts themselves – can be priced into land markets. Severen et al. (2018) find that climate change forecasts are partially priced into land markets, and land values are more strongly related to future climate predictions in counties with higher beliefs in climate change in the US. This study provides an innovative test of how climate change may be priced into vulnerable assets, but certain features of asset markets in developing countries imply that new methods or data may be required to apply these tests in developing countries. These features include the relative thinness of agricultural land markets, as well as transaction costs due to non digitised land records (Beg 2022).
In conclusion, it is worth noting that the bulk of the literature finds large negative impacts of warming and lower rainfall in developing countries with limited adaptation. This indicates that policy interventions to facilitate adaptation will likely be required. In the next section, we review the literature on adaptation responses.
References
Adhvaryu, A, N Kala, and A Nyshadham (2020), "The Light and the Heat: Productivity Co-benefits of Energy-saving Technology," Review of Economics and Statistics, 102(4): 779-792.
Baez, J E, and I V Santos (2007), "Children’s Vulnerability to Weather Shocks: A Natural Disaster as a Natural Experiment," Social Science Research Network, New York: 1-28.
Banerjee, R, and R Maharaj (2020), "Heat, Infant Mortality, and Adaptation: Evidence from India," Journal of Development Economics, 143: 102378.
Beg, S (2022), "Digitization and Development: Property Rights Security, and Land and Labour Markets," Journal of the European Economic Association, 20(1): 395-429.
Bento, A, N S Miller, M Mookerjee, and E R Severnini (2020), “A Unifying Approach to Measuring Climate Change Impacts and Adaptation,” National Bureau of Economic Research, w27247.
Blakeslee, D, and R Fishman (2018), "Weather Shocks, Agriculture, and Crime Evidence from India," Journal of Human Resources, 53(3): 750-782.
Burgess, R, O Deschênes, D Donaldson, and M Greenstone (2017), "Weather, Climate Change and Death in India," University of Chicago.
Burke, M, and K Emerick (2016), "Adaptation to Climate Change: Evidence from US Agriculture," American Economic Journal: Economic Policy, 8(3): 106-140.
Burke, M, S M Hsiang, and E Miguel (2015), "Climate and Conflict," Annu. Rev. Econ., 7(1): 577-617.
Caruso, G D (2017), “The Legacy of Natural Disasters: The Intergenerational Impact of 100 Years of Disasters in Latin America,” Working Paper.
Colmer, J (2021), "Temperature, Labour Reallocation, and Industrial Production: Evidence from India," American Economic Journal: Applied Economics, 13(4): 101-124.
Deschênes, O, and M Greenstone (2007), "The Economic Impacts of Climate Change: Evidence from Agricultural Output and Random Fluctuations in Weather," American Economic Review, 97(1): 354-385.
Deschênes, O, and M Greenstone (2012), "The Economic Impacts of Climate Change: Evidence from Agricultural Output and Random Fluctuations in Weather: Reply," American Economic Review, 102(7): 3761-3773.
Dell, M, B F Jones, and B A Olken (2012), "Temperature Shocks and Economic Growth: Evidence from the Last Half-century," American Economic Journal: Macroeconomics, 4(3): 66-95.
Elliott, R J R, E Strobl, and P Sun (2015), "The Local Impact of Typhoons on Economic Activity in China: A View From Outer Space," Journal of Urban Economics, 88: 50-66.
Fisher, A C, W M Hanemann, M J Roberts, and W Schlenker (2012), "The Economic Impacts of Climate Change: Evidence From Agricultural Output and Random Fluctuations in Weather: Comment," American Economic Review, 102(7): 3749-3760.
Fishman, R, P Carrillo, and J Russ (2019), “Long-term Impacts of Exposure to High Temperatures on Human Capital and Economic Productivity,” Journal of Environmental Economics and Management, 93: 221-238.
Garg, T, M Jagnani, and V Taraz (2020), "Temperature and Human Capital in India," Journal of the Association of Environmental and Resource Economists, 7(6): 1113-1150.
Geruso, M, and D Spears (2018), “Heat, Humidity, and Infant Mortality in the Developing World,” National Bureau of Economic Research.
Guiteras, R (2009), "The Impact of Climate Change on Indian Agriculture," Manuscript, Department of Economics, University of Maryland, College Park, Maryland.
Guha-Sapir, D, R Below, and P Hoyois (2023), "EM-DAT: The CRED/OFDA International Disaster Database", Université Catholique de Louvain – Brussels – Belgium.
Henderson, J V, A Storeygard, and U Deichmann (2017), “Has Climate Change Driven Urbanization in Africa?” Journal of Development Economics, 124: 60–82.
Hsiang, S M, and A S Jina (2014), “The Causal Effect of Environmental Catastrophe on Long-run Economic Growth: Evidence from 6,700 Cyclones,” National Bureau of Economic Research.
Hultgren, A, T Carleton, M Delgado, D R Gergel, M Greenstone, T Houser, S Hsiang, A Jina, R E Kopp, S B Malevich, K McCusker, T Mayer, I Nath, J Rising, A Rode, and J Yuan (2022), "Estimating Global Impacts to Agriculture from Climate Change Accounting for Adaptation," Available at SSRN.
Kala, N (2017), "Learning, Adaptation, and Climate Uncertainty: Evidence from Indian Agriculture," MIT Center for Energy and Environmental Policy Research, Working Paper 23.
Kurukulasuriya, P, N Kala, and R Mendelsohn (2011), "Adaptation and Climate Change Impacts: A Structural Ricardian Model of Irrigation and Farm Income in Africa," Climate Change Economics, 2(2): 149-174.
Lemoine, D (2018), “Estimating the Consequences of Climate Change From Variation in Weather,” National Bureau of Economic Research, No. w25008.
Liu, M, Y Shamdasani, and V Taraz (2023), "Climate Change and Labour Reallocation: Evidence From Six Decades of the Indian Census," American Economic Journal: Economic Policy, 15(2): 395-423.
Maccini, S, and D Yang (2009), "Under the Weather: Health, Schooling, and Economic Consequences of Early-life Rainfall," American Economic Review, 99(3): 1006-1026.
McGuirk, E F, and N Nunn (2021), "Transhumant Pastoralism, Climate Change, and Conflict in Africa," Working Paper.
Mendelsohn, R, W D Nordhaus, and D Shaw (1994), "The Impact of Global Warming on Agriculture: A Ricardian Analysis," The American Economic Review: 753-771.
Miguel, E (2005), "Poverty and Witch Killing," The Review of Economic Studies, 72(4): 1153-1172.
Pelli, M, J Tschopp, N Bezmaternykh, and K Eklou (2023), "In the Eye of the Storm: Firms and Capital Destruction in India," Journal of Urban Economics, 134: 103529.
Rosenzweig, C, J Elliott, D Deryng, A C Ruane, C Müller, A Arneth, K J Boote, C Folberth, M Glotter, N Khabarov, et al. (2014), "Assessing Agricultural Risks of Climate Change in the 21st century in a Global Gridded Crop Model Intercomparison," Proceedings of the National Academy of Sciences, 111(9): 3268-3273.
Rosenzweig, M, and C R Udry (2013), “Forecasting Profitability,” National Bureau of Economic Research, No. w19334.
Santangelo, G (2019), "Firms and Farms: The Local Effects of Farm Income on Firms’ Demand," Working Paper.
Severen, C, C Costello, and O Deschenes (2018), "A Forward-Looking Ricardian Approach: Do Land Markets Capitalize Climate Change Forecasts?" Journal of Environmental Economics and Management, 89: 235-254.
Sekhri, S, and A Storeygard (2014), "Dowry deaths: Response to weather variability in India" Journal of Development Economics, 111: 212-223.
Shah, M, and B M Steinberg (2017), "Drought of Opportunities: Contemporaneous and Long-term Impacts of Rainfall Shocks on Human Capital," Journal of Political Economy, 125(2): 527-561.
Shrader, J (2017), "Expectations and Adaptation to Environmental Risks," Working Paper.
Zheng, P, O Deschenes, K Meng, and J Zhang (2018), “Temperature effects on productivity and factor reallocation: Evidence from a half million Chinese manufacturing plants,” Journal of Environmental Economics and Management, 88:1-17.
Contact VoxDev
If you have questions, feedback, or would like more information about this article, please feel free to reach out to the VoxDev team. We’re here to help with any inquiries and to provide further insights on our research and content.