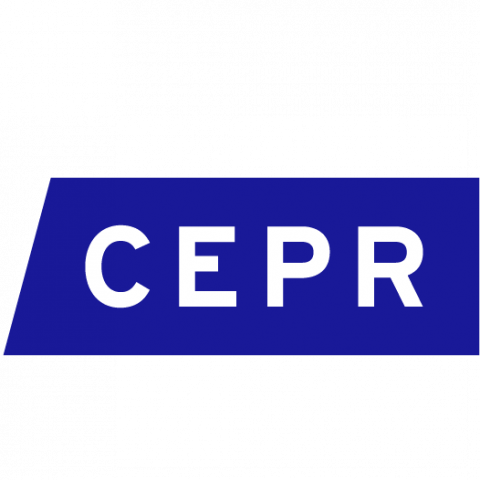
How can we estimate state presence in areas where direct measurements are lacking? New research offers a solution to measuring state presence using machine learning techniques.
The ability of states to exercise authority often varies considerably within their borders. This uneven reach of the state has important consequences for economic and political life. For instance, it is well-known that rebel groups tend to form and operate in remote areas where state authorities are weaker (Fearon and Laitin 2003). Yet, we lack empirical measurements that can inform us on states’ ability to exercise authority at a fine-grained spatial level.
A machine learning solution to mapping state presence
In our recent paper (Agneman et al. 2025), we propose a machine learning-based solution that integrates the detailed information from survey data (Fergusson et al. 2022) with geographically comprehensive indicators of state presence (Müller-Crepon et al. 2021). The approach builds on a three-step strategy.
First, we estimate an index that captures state presence as experienced by local residents. The data is drawn from two rounds of the Afrobarometer (BenYishay et al. 2017), in which individuals are asked about the state’s ability to uphold law and order in their area of residence. We aggregate the information from six survey questions that capture different dimensions of state presence using a principal component analysis, thereby obtaining a ‘ground-truth’ measure of experienced state presence in a subset of African cities and villages (Figure 1).
Figure 1: Experienced state presence at the enumeration area-level
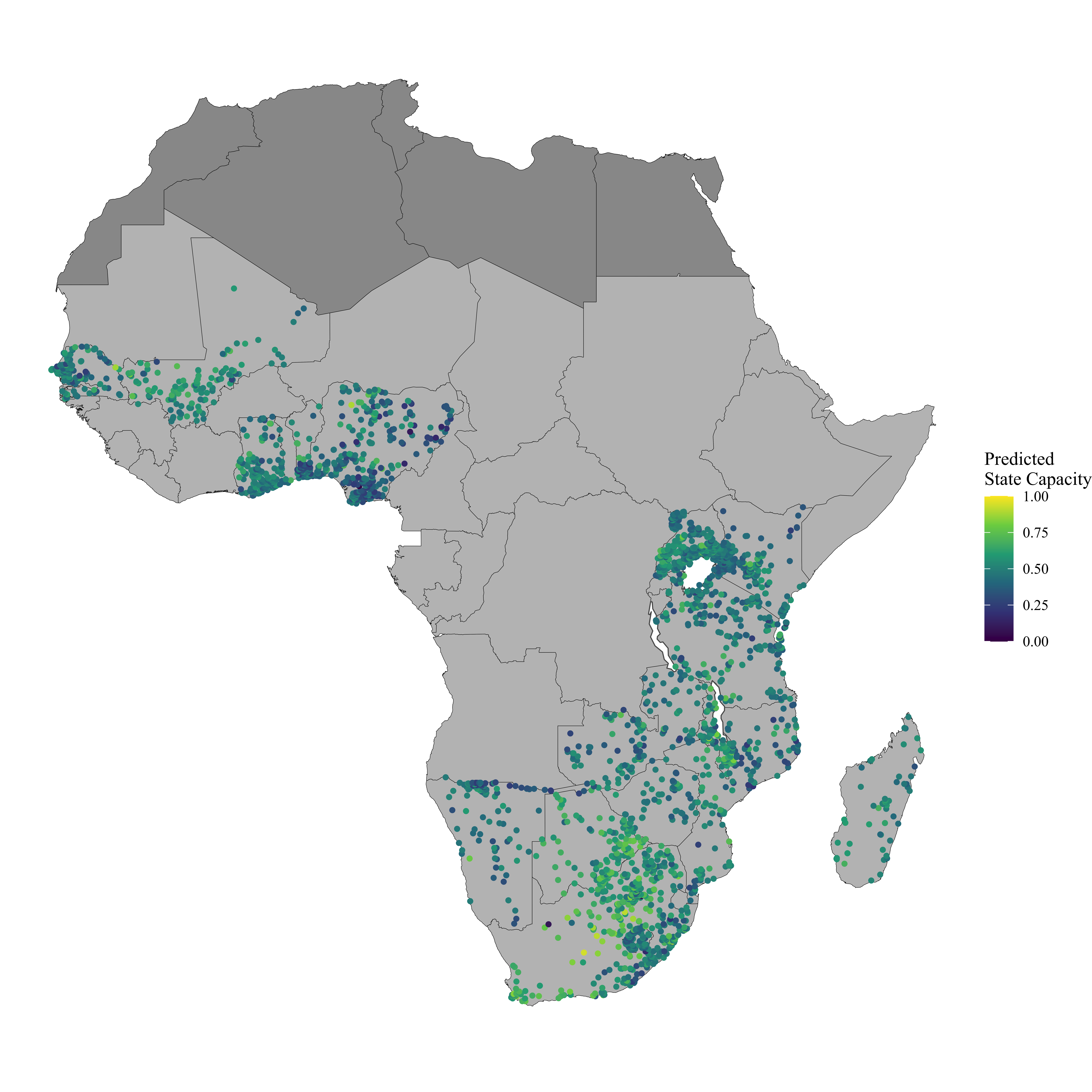
Second, we link the index of experienced state presence with a range of data that should theoretically associate with state presence, such as altitude (Scott 2009), road infrastructure (Müller-Crepon et al. 2021), and territorial control at the state level (Berman et al. 2017). These variables, visualized in Figure 2, have served as proxies for state presence in earlier research. We use a machine learning algorithm to predict experienced state presence using these variables as predictors. The algorithm learns how the variables interact to predict experienced state presence and captures non-linear relationships through piecewise linear functions.
Figure 2: The predictors mapped
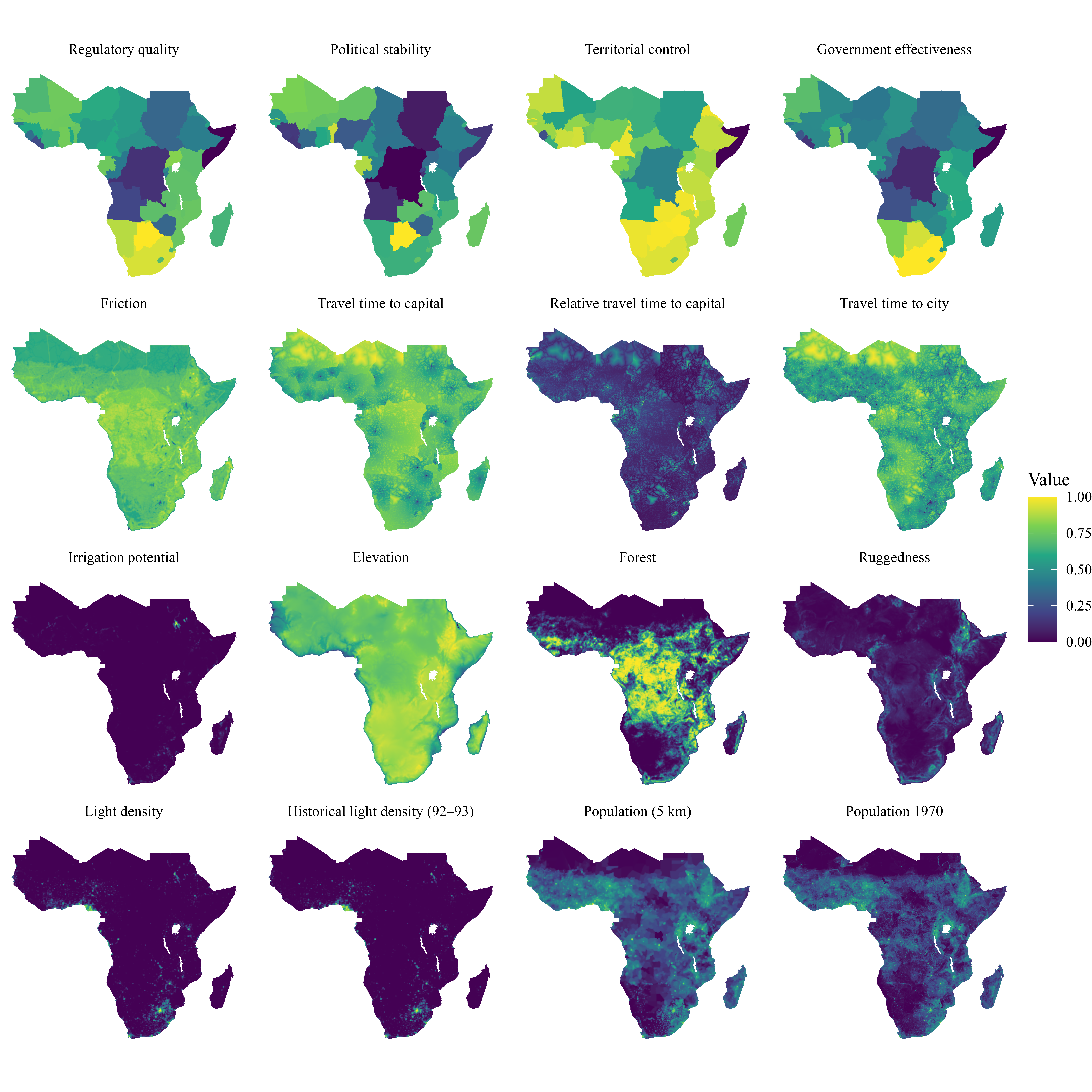
Third, we use the model’s predictions to extrapolate a spatially fine-grained measure of state presence across Sub-Saharan Africa (Figure 3). This measure is the model’s best estimate of state authority in a given area based on the values of input data. The machine learning algorithm determines the weight of each predictor, assigning greater weight to variables that are highly predictive of experienced state presence. By extrapolating these predictions, we can generate a measure of state presence in areas where survey data is unavailable.
Figure 3: Predicted local state presence
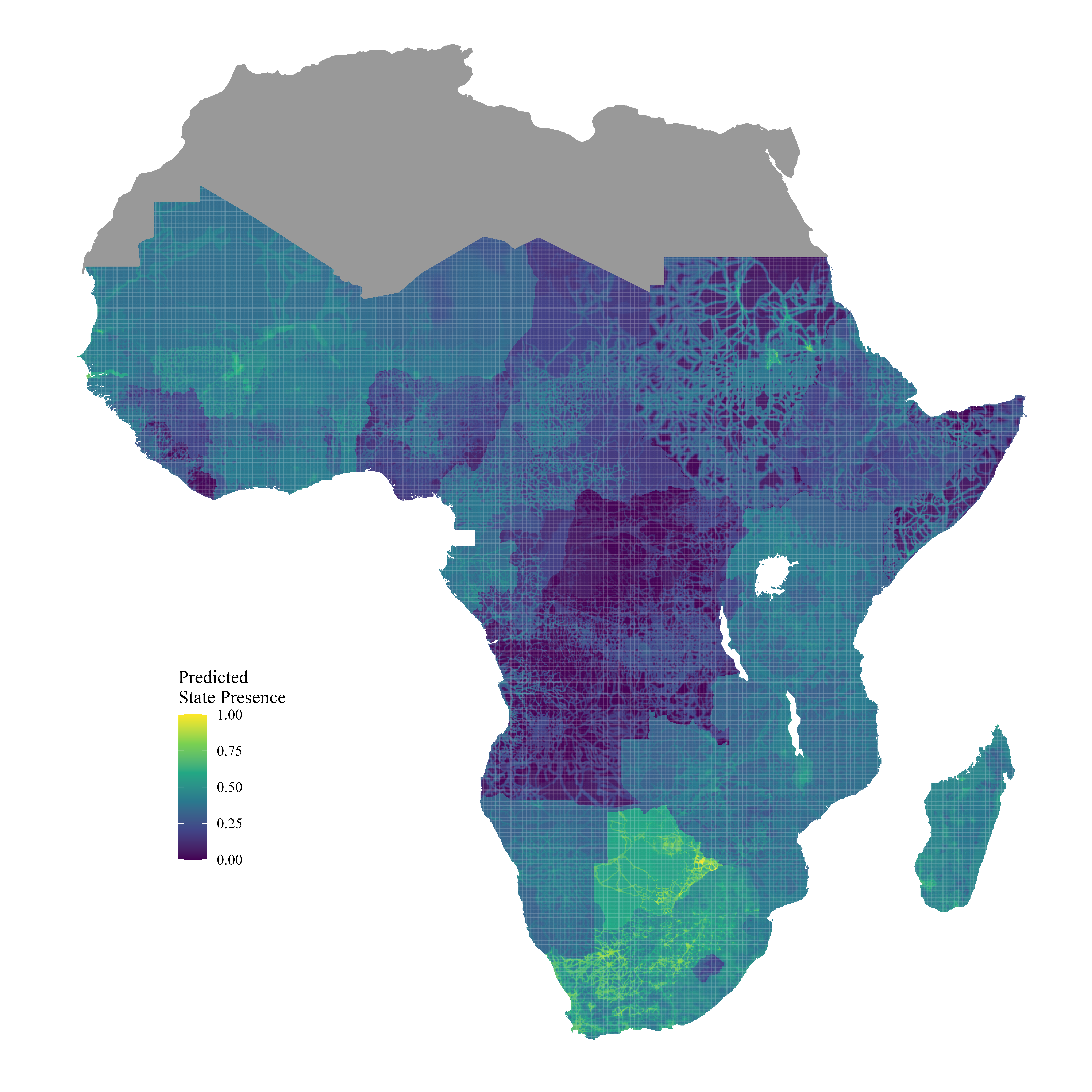
We conduct multiple exercises to ensure that the measure accurately reflects the underlying concept:
- We find that predicted state presence correlates positively with the number of government employees at the district level in Ghana.
- Our measure is shown to be strongly correlated with an indicator of administrative capacity developed by Lee and Zhang (2017).
- We demonstrate cross-sectional and inter-temporal validity using Afrobarometer data from countries and survey rounds not included in the machine learning model.
This exercise documents strong correlations with ‘objective’ indicators of state presence, such as post offices, sewage systems, and tarred roads.
Finally, we put the data to use by revisiting the oil-conflict hypothesis. To this end, we link predicted state presence with data on oil deposits from Rystad Energy (Rystad Energy Data Bank 2020) and oil-related conflict from the Armed Conflict Location and Event Dataset (ACLED) database (Raleigh et al. 2017). We then employ our index of local state presence as a moderating variable in panel fixed-effects regressions with oil conflict as the outcome. In line with our expectations, we find that local state presence mitigates the risks of oil conflicts. In fact, while oil wealth triggers oil conflicts in areas of low state presence, we find no significant results in areas of high state presence.
Policy implications
Our paper presents a new approach to measuring state presence at the spatial level where events actually unfold—at the local level (Berman et al. 2017). As shown in this paper, such a measure is important if we want to understand how conflict dynamics may vary between contexts. Similarly, a range of other development outcomes—such as health, political participation, and climate vulnerability—depend on local state presence. To support research in those and other areas, our measure of local state presence is made publicly available here.
References
Agneman, G, A BenYishay, D Runfola, and R D Manners (2025), “The uneven reach of the state: A novel approach to mapping local state presence,” Journal of Development Economics, 103453.
Berman, N, M Couttenier, D Rohner, and M Thoenig (2017), “This mine is mine! How minerals fuel conflicts in Africa,” American Economic Review, 107(6): 1564–1610.
BenYishay, A, D Runfola, T Goodman, and R D Manners (2017), “Geocoding Afrobarometer Rounds 1–6: Methodology & data quality,” AidData.
Depetris-Chauvin, E (2015), “State history and contemporary conflict: Evidence from Sub-Saharan Africa,” Unpublished manuscript.
Fearon, J D (2005), “Primary commodity exports and civil war,” Journal of Conflict Resolution, 49(4): 483–507.
Fearon, J D, and D D Laitin (2003), “Ethnicity, insurgency, and civil war,” American Political Science Review, 97(1): 75–90.
Fergusson, L, C A Molina, and J A Robinson (2022), “The weak state trap,” Economica, 89(354): 293–331.
Lee, M M, and N Zhang (2017), “Legibility and the informational foundations of state capacity,” The Journal of Politics, 79(1): 118–132.
Müller-Crepon, C, P Hunziker, and L-E Cederman (2021), “Roads to rule, roads to rebel: Relational state capacity and conflict in Africa,” Journal of Conflict Resolution, 65(2–3): 563–590.
Raleigh, C, A Linke, H Hegre, and J Karlsen (2010), “Introducing ACLED: An Armed Conflict Location and Event Dataset,” Journal of Peace Research, 47(5): 651–660.
Rystad Energy (2020), "Rystad Energy Data Bank."
Scott, J C (2009), "The art of not being governed: An anarchist history of upland Southeast Asia," Yale University Press.