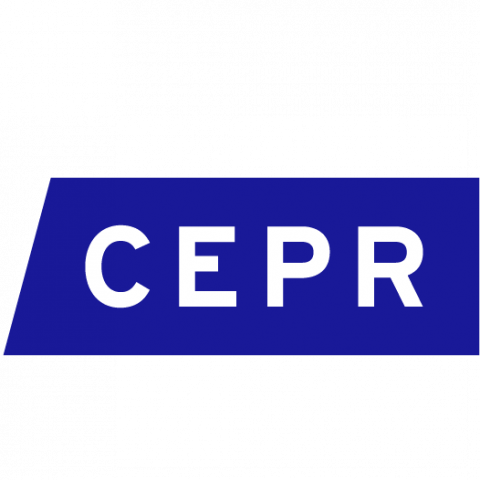
When governments harness public budget data and machine learning, they can better predict the incidence of local government corruption.
Corruption remains a critical governance issue globally, imposing significant costs on society through inefficiency, inequality, and loss of public trust (Morris and Klesner 2010, Ferraz et al. 2012, Colonelli et al 2022). One central difficulty in combating corruption is accurately identifying corrupt practices, which are inherently concealed by corrupt actors. Traditionally, corruption detection relies on human audits and whistleblower reports, which are both costly and subject to selection biases and inefficiencies.
How machine learning can improve corruption detection and prevention
A promising approach to enhancing the detection and prevention of corruption is to adopt recent advances in machine learning (ML) and apply them to extensive data collected by public institutions. The underlying intuition of this method is clear: corrupt activities typically leave subtle, but distinct, traces in administrative behaviour. For example, by thoroughly analysing administrative records such as municipal budgets, advanced ML algorithms can identify distinct patterns indicative of corruption—patterns that human auditors might miss or be unable to detect due to cognitive biases, limited resources, or sheer complexity.
In our research (Ash et al. 2025), we explore this approach using Brazilian municipal budget data. Using the gradient-boosted classification algorithm XGBoost (Chen et al. 2016), we trained a predictive model to identify corruption based solely on municipal budget allocations and financial practices. Our dataset comprised nearly 800 detailed budget variables for thousands of municipalities, matched with verified corruption outcomes from random audits conducted by federal authorities.
Figure 1: Performance comparison in detecting corruption across machine learning methods
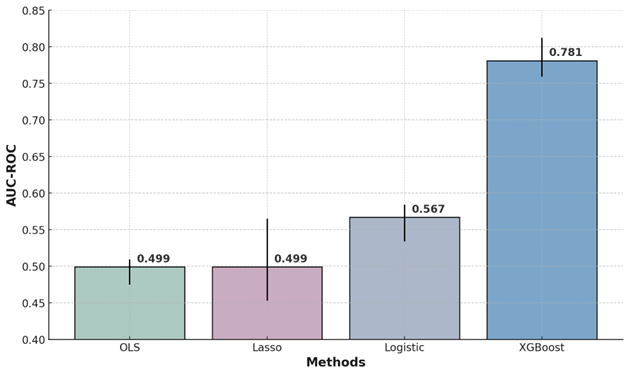
The performance of XGBoost in predicting corruption was robust and substantially outperformed traditional methods such as ordinary least squares (OLS), Lasso regression, and logistic regression. Specifically, our XGBoost model achieved an AUC-ROC of approximately 0.78, significantly higher than traditional methods, which hovered near random guessing (around 0.5 to 0.57). Practically, an AUC-ROC of 0.78 means that if we randomly select one corrupt entity and one non-corrupt entity from our dataset, there is a 78% chance that the model will correctly rank the corrupt entity as having a higher probability of corruption. This strong predictive performance demonstrates the feasibility and potential accuracy of ML approaches in detecting corruption through administrative data.
The implications of machine learning for research on corruption
Beyond methodological innovation, the predictive model presents new opportunities for academic research. Traditionally constrained by limited corruption data—often available only through infrequent and expensive audits—scholars now can generate synthetic corruption measures at scale. This allows for robust empirical analyses of corruption determinants, effects, and dynamics over larger samples and across broader geographic and temporal contexts. For example, we extend the work of Avis et al. (2018), providing further evidence on the effects of audits on corruption. Our findings suggest that auditing significantly reduces budgeting behaviour predictive of corruption, particularly after corruption is initially detected.
Figure 2: Effects of audits on corruption, measured using machine learning
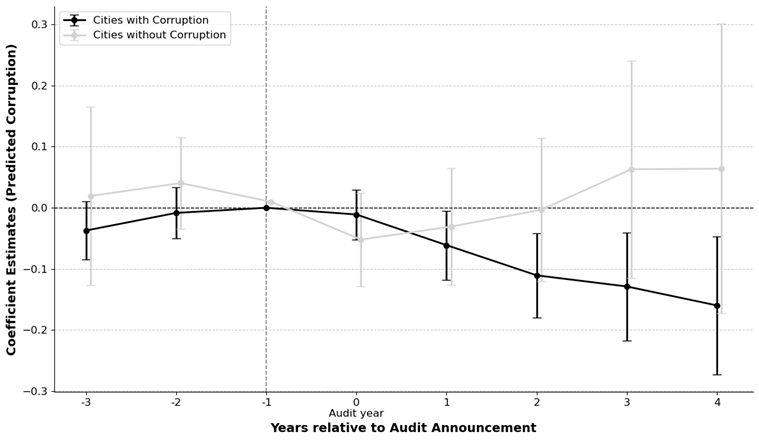
The implications of machine learning for anti-corruption policy
Our predictive approach holds significant practical potential to enhance policy effectiveness. Corruption audits are conducted randomly, limiting their efficiency in terms of detection. In our research, we show that by implementing a targeted auditing policy based on our ML-generated corruption risk scores, authorities could substantially improve detection rates. Our policy simulations indicate that, compared to random audits, targeted audits informed by ML predictions could increase the probability of receiving an audit for a corrupt administration by approximately 2.7 times—from 2.8% under random audits to 7.6% under targeted audits.
The machine-learning approach to corruption policy is applicable to jurisdictions worldwide. The necessary ingredients are ground-truth labels for corruption (e.g. the results of corruption audits) and associated predictive features (e.g. data on the budget accounts). Other equivalent data would also be sufficient: for example, getting corruption labels from the results of judicial proceedings, or getting predictive variables from the accounts of firms, rather than governments. In Brazil, a helpful institutional feature is that the audits are randomly assigned, making the model’s predictions generalisable to all municipalities. In the case of models trained on labels from non-random audits, the predictions will likely still be helpful, but perhaps not as robustly predictive as is the case in Brazil.
Figure 3: Effectiveness of targeting audits using machine learning
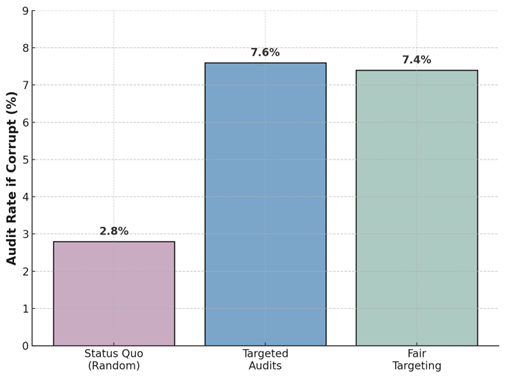
Notably, the adoption of ML-driven audits must confront concerns about potential biases, especially regarding political fairness. One impediment to adopting algorithmic targeting of audits is the potential perception that these algorithms are biased toward one political party or another. Our study explicitly addresses this issue. We show that even after adjusting for potential political biases—specifically, equalising audit rates across political parties—targeted audits remain highly effective, retaining substantial improvements over random auditing. More specifically, with this fair targeting, the ML predictions could still increase the chance of receiving an audit for a corrupt administration from 2.8% to 7.4% (rather than 7.6% without the fairness adjustment). Overall, ML predictions offer a powerful, politically feasible method for corruption detection.
Nevertheless, implementing ML-driven auditing in practice involves important considerations beyond these initial results. A crucial open question is the dynamic effect of deploying such predictive tools. Administrators may adapt their strategies over time to evade detection, potentially reducing the model’s accuracy and overall effectiveness. These adaptive behaviours necessitate ongoing model updating and further research into how ML predictions influence corrupt actors' incentives and actions.
Conclusion: Machine learning as a tool for corruption detection and deterrence
In sum, our work demonstrates that ML can significantly improve corruption detection by analysing public budget records. Our predictive measure not only expands academic research opportunities but also provides policymakers with a tool to improve corruption detection and deterrence. While challenges remain, particularly regarding dynamic behavioural responses, the promise of ML-driven anti-corruption policies warrants further exploration and consideration by governments and international organisations committed to reducing corruption.
References
Ash, E, S Galletta, and T Giommoni (forthcoming), “A machine learning approach to analyze and support anti-corruption policy,” American Economic Journal: Economic Policy.
Avis, E, C Ferraz, and F Finan (2018), “Do government audits reduce corruption? Estimating the impacts of exposing corrupt politicians,” Journal of Political Economy, 126(5): 1912–1964.
Chen, T, and C Guestrin (2016), “XGBoost: A scalable tree boosting system,” in Proceedings of the 22nd ACM SIGKDD International Conference on Knowledge Discovery and Data Mining, 785–794.
Colonnelli, E, M Prem, F Teso, and F Toffano (2022), “Revealing corruption: Firm and worker level evidence from Brazil,” Journal of Financial Economics, 143(3): 1097–1119.
Ferraz, C, F Finan, and D B Moreira (2012), “Corrupting learning: Evidence from missing federal education funds in Brazil,” Journal of Public Economics, 96(9–10): 712–726.
Morris, S D, and J L Klesner (2010), “Corruption and trust: Theoretical considerations and evidence from Mexico,” Comparative Political Studies, 43(10): 1258–1285.