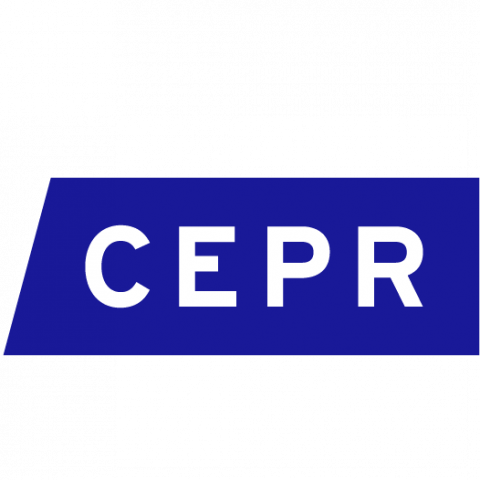
Many agricultural technologies are extremely sensitive to local circumstances: nutrients, moisture, soil quality, altitude, temperatures, soil structure, topography and solar energy are all required in appropriate proportions and timing for crops and animal husbandry, and farmers face different threats from diseases and pests (Evenson and Westphal 1995, Moscona and Sastry 2022). Overlaid on these natural conditions is heterogeneity in infrastructure and market access, and hence in the prices of inputs and outputs, again both over time and space. Even in agricultural regions outside Africa with well-developed agricultural technology use we see fine geographical variation in optimal technology choices: in the American state of Illinois, for example, optimal maize varieties differ at the scale of a few dozen miles (for example, see https://burrusseed.com/product-selection-guide/).
There could be several implications of this heterogeneity. One, of course, is that some technologies may simply not be profitable everywhere. But there are additional implications: e.g. it might be hard to learn from one’s neighbours if they have different conditions to your own, and there might be limited incentives for R&D of technologies if they have small markets and are only relevant for a small segment of the population. For example, evidence suggests that many agricultural technologies are developed by and most appropriate for high-income countries. This mismatch limits the transfer of technologies to low-income countries that have different pests and pathogens and reduces global crop production (Moscona and Sastry 2022).
Heterogeneity may be key to understanding technological stagnation in African agriculture more broadly. For Kenya, Suri (2011) shows large heterogeneity in both gross and net returns to hybrid seed and fertiliser. Indeed, the extent of heterogeneity appears to be far larger in sub-Saharan Africa than in temperate regions. Claassen and Just (2011) study the variance of log yield across farms in the United States: they find that the 95th percentile of corn yield is 190% larger than the 5th percentile yield. For comparison, the 95-5 ratio for Uganda is 9,304% and for Tanzania 2,558% (Gollin and Udry 2021). A substantial share of this variation is likely due to heterogeneity in soil, moisture, temperature and other dimensions of growing conditions over space. But the heterogeneity is not fully exogenous: some technologies might reduce this heterogeneity — e.g. irrigation lowers the degree to which yields vary with rainfall. The exceptional degree of heterogeneity in Africa is at least in part a consequence of the 18th to 20th century history of low population density on most of the continent (itself caused in part by the slave trade and associated violence and political disruptions as discussed in Manning (2014)). The low population density increases infrastructure costs, so that farmers rely on rainfall for irrigation and on periodic fallowing (rather than fertiliser) to maintain soil fertility (Boserup 1965). Agricultural production therefore is more tightly connected to local conditions such as rainfall realisations, plot-level availability of soil nutrients and the existence (or not) of a nearby road and/or market. The implications of such heterogeneity can be far reaching, not just in explaining the lack of adoption, but also in underscoring the challenges of developing new agricultural technologies and designing policy that ultimately improves yields.
Soil and land quality
The nutrient composition, physical properties and biochemistry of the soil affects the profitability of any given seed, fertiliser or other chemical input, or other agronomic practice. Sanchez (2019) provides a comprehensive review of soil properties and soil management across the world, highlighting the extreme heterogeneity in soil quality and the importance of the interaction of nutrients and water availability. For example, the maize yield response to fertiliser is strongly dependent on sandiness and on soil carbon content and thus farmers’ use of fertiliser responds directly to soil conditions (Marenya and Barrett 2009a, 2009b, Burke et al. 2020). These land characteristics vary in important ways across communities, at scales as small as hundreds of metres — Hengl et al. (2021) show maps of data collected by the International Soil Reference and Information Centre (ISRIC), displaying differences in the acidity of soil at a resolution of 30 metres. Researchers have shown and farmers have described substantial variation in soil characteristics within farms, and farmers adjust production decisions to accommodate that variation (for example, Tjernström et al. 2015). Soil quality is not fixed, but evolves over time in response to farmer actions, weather shocks and environmental influences including seed dispersal, erosion, rainfall, and the movement of pests.
Given large variations in soil quality across even small geographic areas, optimal technology choices will vary between plots or even on a specific plot over time. For example, Harou et al. (2022) show that in rural Tanzania, within-village variation accounts for almost one-third of overall variation across the district in several key soil nutrients, and that recommended amounts and types of fertiliser vary significantly within villages. Farmers who were provided with plot-specific recommendations for appropriate fertiliser use (along with vouchers for reduced cost access to inputs) were more likely to apply the recommended fertiliser, and increased yields by over 150% relative to the control group. This work highlights both spatial variation in soil characteristics (lots of variation within villages) and faulty information. Government recommendations do not address sulphur and about 95% of plots in the entire sample are sulphur-deficient; farmers that receive the information plus the vouchers address the sulphur deficiency but they do not act on the more nuanced, plot-specific limitations that vary within villages. Abay et al. (2022) show that farmers in Ethiopia do not apply the correct types or amounts of fertiliser that their plots require, lowering yields and partially explaining the heterogeneity in returns to fertiliser. Additionally, Kurdi et al. (2020) find that incentives can be perverse - a fertiliser subsidy for nitrogen fertilisers in Egypt led to the overapplication of nitrogen fertiliser, which could then adversely affect soil, water, and environmental health. Berazneva et al. (2023) experimentally test the relationship between within-village soil heterogeneity and farmer investment in soil testing across villages in Malawi. The experiment randomly varies the plot to be tested across village residents and shows that farmers contribute more to the testing of plots that they perceive as similar to their own. Free riding occurs when more farmers in a village have soils of the same type.
Heterogeneity in weather
The returns to investment in cultivation in sub-Saharan Africa (and South Asia) can vary widely, depending upon the local weather (Rosenzweig and Udry 2020). As a recent example, McCullough et al. (2018) use an experimental crop trial meta-database to show that the profitability of adopting fertiliser varies strongly with weather outcomes. This temporal heterogeneity makes the adoption decisions of farmers much more difficult; it also demands a longer-term perspective for those who seek to understand these adoption decisions.
There has been some investment to develop and disseminate crop varieties that are more resistant to poor weather realisations, and in particular to varieties that may outperform the alternatives during poor weather realisations. This is an exciting area of varietal improvement, though such varieties are still few and far between. Examples of such varieties are Swarna Sub-1 flood resistant rice in India (Emerick et al. 2016) and drought resistant maize (Boucher et al. forthcoming).
Heterogeneity in access to markets
Scarce and low-quality road infrastructure, together with imperfect competition in transportation, similarly generate large variation across space in both input and output prices. These high transportation costs interact with a spatially dispersed population of farmers to produce markets for agricultural output and inputs that are far from a perfectly competitive benchmark (Bergquist and Dinerstein 2020), limiting technology adoption and farm productivity (Aggarwal et al. 2022, Minten et al. 2013). The traders to whom farmers sell (often at farm gate) are not competitive, further affecting output prices (for example, Casaburi et al. 2013). The consequent price mark-ups also vary across space. Some countries have a policy of uniform national prices of some inputs; such policies are problematic given high transportation costs because they can lead to considerable spatial variation in access to these inputs and hence in their returns and adoption, as Suri (2011) shows for Kenya.
These high, heterogeneous, and variable trade costs interact with the adoption of new technologies in specific ways; for example, subsidies for fertiliser only raise farmer incomes when trade costs are low (Porteous 2020). Kumar et al. (2023) also show that costs for adopting technologies, even with the provision of subsidies, is more expensive for rural farmers who need to travel and may need to buy from specific retailers. They examine the Malawi Farm Input Subsidy Programme (FISP) and find that the subsidy reduces the spatial cost gradient, but does not eliminate it.
Pervasive heterogeneity and technology adoption
This pervasive spatial and temporal heterogeneity presents challenges, both for African farmers attempting to make optimal technology decisions and for researchers seeking to understand farmers’ technological choices. A technology that is profitable on one set of farms in a particular year may fail on other nearby farms or in other seasons, limiting the extent of learning and the duration needed for learning about benefits to be sufficient to induce adoption.
Agricultural technologies vary in the degree and nature of their sensitivity to local circumstances. The profitability of adopting mechanical field preparation in savannah regions (that is, a grassland with numerous but widely spaced trees), for example, depends on factor prices and topography, but not as strongly on local variations in soil characteristics. The profitability of adopting improved storage technologies depends on prices and local insect populations, but not as much on topography. Innovations tied more closely to the biological and physical characteristics of a plot, like chemical inputs and seed varieties, exhibit the most heterogeneity in returns (for example, Harou et al. 2022).
Farmers presented with an opportunity to adopt a new agricultural technology must translate information they receive regarding the performance of the technology in specific circumstances to its likely performance on their own farms. Understanding how farmers make this translation remains incomplete. Information from extension agents and expert advice can be effective, but some farmers place more weight in learning from others who are more like themselves (for example, Munshi 2004, BenYishay et al. 2019).
Heterogeneity has implications for the optimal supply of agricultural innovations as well, because the circumstantial sensitivity of many innovations limits the extent of their potential impact, reducing the incentives for private research. Heterogeneity can also make it costly and complex to scale extension or for agri-dealers to stock an appropriate range of locally-relevant technologies. In addition, any cost-benefit analysis for public support of research into new agricultural technologies needs to take the more restricted impact into account.
The RCT results reported in Bird et al. (2022) show that improved maize varieties locally adapted for a small, “niche,” agro-ecology in Kenya’s mid-altitude zone indeed offer substantial yield and economic gains above and beyond those offered by hybrids bred for broader market segments. These authors argue that this lack of adaptive local breeding likely explains the very low uptake of improved seeds in this agro-ecological niche (25% in the mid-altitude region versus 80% in Kenya’s larger agro-ecological regions). They also argue that the cost-structure of large seed breeders and the capital constraints of local seed companies present an organisational challenge to the successful creation of varieties of Africa’s many heterogeneous zones.
References
Abay, K A, M H Abay, M Amare, G Berhane, and E Aynekulu (2022), “Mismatch between soil nutrient deficiencies and fertilizer applications: Implications for yield responses in Ethiopia”, Agricultural Economics, 53(2): 215-230.
Aggarwal, S, B Giera, D Jeong, J Robinson, and A Spearot (2022), “Market Access, Trade Costs, and Technology Adoption: Evidence from Northern Tanzania”, Review of Economics and Statistics, forthcoming.
BenYishay, A and A M Mobarak (2019), "Social Learning and Incentives for Experimentation and Communication", The Review of Economic Studies, 86(3): 976-1009.
Bergquist, L F and M Dinerstein (2020), "Competition and Entry in Agricultural Markets: Experimental Evidence from Kenya", American Economic Review, 110(12): 3705-47.
Bird, S S, M R Carter, T J Lybbert, M Mathenge, T Njagi, and E Tjernström (2022), “Filling a Niche? The Maize Productivity Impacts of Adaptive Breeding by a Local Seed Company in Kenya”, Journal of Development Economics, 157: 102885.
Boserup, E (1965), The Conditions of Agricultural Growth: The Economics of Agrarian Change under Population Pressure, London: G Allen & Unwin.
Boucher, S, M R Carter, T Lybbert, J Malacarne, P Marenya and L Paul (forthcoming), “Bundling Genetic and Financial Technologies for More Resilient and Productive Small-scale Agriculture,” Economic Journal.
Burke, W J, S S Snapp and Thomas S Jayne (2020), "An In‐depth Examination of Maize Yield Response to Fertilizer in Central Malawi Reveals Low Profits and Too Many Weeds", Agricultural Economics, 51(6): 923-940.
Casaburi, L, R Glennerster, and T Suri (2013), “Rural Roads and Intermediated Trade: Regression Discontinuity Evidence from Sierra Leone”, Available at SSRN 2161643.
Claassen, R and R E Just (2011), “Heterogeneity and Distributional Form of Farm-Level Yields”, American Journal of Agricultural Economics, 93(1): 144–60.
Emerick, K, A de Janvry, E Sadoulet and M H. Dar (2016), "Technological Innovations, Downside Risk, and the Modernization of Agriculture.", American Economic Review, 106(6): 1537-61.
Evenson, R E and L E Westphal (1995), "Technological change and technology strategy.", Handbook of Development Economics, 3: 2209-2299.
Gollin, D and C Udry (2021), "Heterogeneity, measurement error, and misallocation: Evidence from African agriculture.", Journal of Political Economy, 129(1): 000-000.
Harou, A P, M Madajewicz, H Michelson, C A Palm, N A. Amuri, C Magomba, J M Semoka, K Tschirhart and R Weil (2022), "The joint effects of information and financing constraints on technology adoption: Evidence from a field experiment in rural Tanzania.", Journal of Development Economics, 155. p.102707.
Hengl, T, M A E Miller, J Križan, K D Shepherd, A Sila, M Kilibarda, O Antonijević (2021), "African soil properties and nutrients mapped at 30 m spatial resolution using two-scale ensemble machine learning.", Scientific Reports, 11(1): 1-18.
Jayne, T S, J Chamberlin, S Holden, H Ghebru, J Ricker-Gilbert and F Place (2021), "Rising land commodification in sub-Saharan Africa: Reconciling the diverse narratives." Global Food Security, 30: 100565.
Kumar, N, R Kapoor, S Aggarwal, D Jeong, D S Park, J Robinson and A Spearot (2023), “Going the Extra Mile: Farm Subsidies and Spatial Convergence in Agricultural Input Adoption”, NBER Working Paper no. w31704.
Kurdi, S, M Mahmoud, K A Abay and C Breisinger (2020), “Too much of a good thing? Evidence that fertilizer subsidies lead to overapplication in Egypt”, (Vol. 27). Intl Food Policy Res Inst.
Manning, P (2014), “African Population, 1650-2000: Comparisons and Implications of New Estimates” in Akyeampong, E, R Bates, N Nunn and J Robinson, eds. Africa’s Development in Historical Perspective. Cambridge: Cambridge University.
Marenya, P P and C B Barrett (2009a), “State‐conditional fertilizer yield response on western Kenyan farms.” American Journal of Agricultural Economics, 91(4): 991-1006.
Marenya, P P and C B Barrett (2009b), “Soil quality and fertilizer use rates among smallholder farmers in western Kenya.” Agricultural Economics, 40(5): 561-572.
McCullough, E B, J D Quinn, A M Simons, L J Verteramo and J Woodard (2018), "Modeling climate smart soil health investments in Sub-Saharan Africa.", Unpublished.
Minten, B, B Koru, and D Stifel (2013), “The Last Mile(s) in Modern Input Distribution: Pricing, Profitability, and Adoption”, Agricultural Economics, 44(6): 629–646.
Moscona, J and K Sastry (2022), “Inappropriate Technology: Evidence from Global Agriculture.”, Working Paper, SSRN: http://dx.doi.org/10.2139/ssrn.3886019.
Munshi, K (2004), "Social learning in a heterogeneous population: technology diffusion in the Indian Green Revolution.", Journal of Development Economics, 73(1): 185-213.
Porteous, O (2020), "Trade and agricultural technology adoption: Evidence from Africa.", Journal of Development Economics, 144: 102440.
Rosenzweig, M R and C Udry (2020), "External validity in a stochastic world: Evidence from low-income countries.", The Review of Economic Studies, 87(1): 343-381.
Sanchez, P A (2019), Properties and Management of Soils in the Tropics. London: Cambridge University Press.
Sheahan, M and C B Barrett (2017), “Ten striking facts about agricultural input use in Sub-Saharan Africa.” Food Policy, 67, 12-25.
Suri, T (2011), "Selection and comparative advantage in technology adoption.", Econometrica, 79(1): 159-209.
Tjernström, E, M Carter and T Lybbert (2015), “The Dirt on Dirt: Soil Characteristics and Variable Fertilizer Returns in Kenyan Maize Systems.”, Working paper, USAID Feed the Future Innovation Lab for Assets and Market Access, Davis, CA.
Contact VoxDev
If you have questions, feedback, or would like more information about this article, please feel free to reach out to the VoxDev team. We’re here to help with any inquiries and to provide further insights on our research and content.