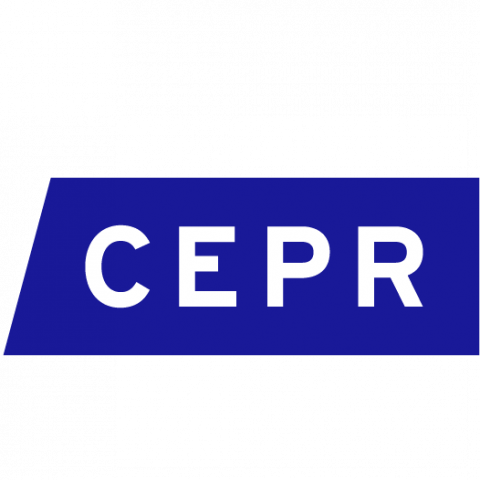
We provide a framework for understanding the range of constraints that account for the stagnation of adoption of productive agricultural technologies in Africa. We highlight some of the more recent studies in African contexts that help us understand why technologies that appear to improve productivity are not developed or adopted. A key source of evidence on the importance of these constraints is provided by the 26 randomised controlled trials (RCTs) across ten African countries supported by the Agricultural Technology Adoption Initiative (Bridle et al. 2019). Broader reviews can also be found in de Janvry et al. (2017), Binswanger-Mkhize and McCalla (2010), Magruder (2018) and Suri and Udry (2021), the last review specifically for the case of Africa. Ultimately, none of these constraints taken alone seem sufficient to explain the low levels of technology adoption – and hence low yields – seen in Africa.
Summary: No single binding constraint
No single constraint explains the low productivity in African agriculture; instead, different combinations of constraints seem to bind for different farmers. As a result, packages of technological, policy and institutional interventions - so-called ‘socio-technical innovation bundles” (Barrett et al. 2022) – may be the most useful approach to encourage the adoption of new technologies and thus improve agricultural productivity. Current knowledge about effective packages of interventions is limited (we return to this in more detail below), though the literature on this is growing fast.
Examples of packages include a programme in Kenya organised by a non-profit called Drumnet that showed that combining training, agricultural credit, improved access to saving, input supply and marketing assistance led to increased adoption of a novel export crop, french bean (and the related inputs) (Ashraf et al. 2009). Also in Kenya, the One Acre Fund’s programme of group lending, crop insurance, regular training, input supply and market facilitation support generated adoption of improved practices, increased use of fertiliser and increased yield and farm profits (Deutschmann et al. 2019). A multi-faceted “economic inclusion” programme in Niger with training, large cash grants, and a set of psycho-social interventions generated substantial increases in improved livestock and the use of fertiliser and phytosanitary inputs (Bossuroy et al. 2022). Mukherjee et al. (2022) find a combination of credit and savings products lead farmers to invest more in farm inputs. Similarly, Deutschmann et al. (2021) find that bundling price premium certainty with training and credit increases adoption of a quality-improving technology in Senegal; Macchiavello and Miquel-Florensa (2019) find that a combination of contractual arrangements resulted in quality upgrading and expanded land under cultivation. Finally, Arouna et al. (2021) test various bundles of contract farming offers, though they find that the simplest option offering just price certainty works just as effectively as versions that bundle price certainty with extension services and/or loans.
All this said, multifaceted programmes are far from a silver bullet. The Drumnet export-promotion programme in Kenya, for example, collapsed after a year when farmers were unable to meet EU production requirements. Other research efforts that have attempted to link buyers with specific quality requirements to smallholder farmers, under the theory that this could stimulate investment in provision of training and input credit, were unable to do so due to farmers’ inability to reliably deliver the volume of output required (Hoffmann et al. 2018, Magnan et al. 2021), a challenge inherent in Africa’s predominantly rainfed production systems. Some earlier integrated public programmes were costly failures, like the Integrated Rural Development Programmes implemented in the 1970s-1980s (Chambers 2014), and some community-driven rural development programmes had minimal effects (Appiah et al. 2020). Moreover, several approaches to input intensification, while increasing crop yields, generated programme costs that often exceeded the value of additional crop output (Jayne et al. 2018). Could the scarce resources involved have raised agricultural productivity more cost-effectively had they been allocated differently? We still have limited understanding of what programme elements are essential in which environments, and less still regarding the costs of tailoring programmes to these varying environments.
Farmers do show an ability to overcome constraints when the technology is sufficiently productive (i.e. increases productivity enough) and hence actually profitable (Suri 2011). Many examples exist of farmers adopting a technology in particular contexts, such as cocoa in Ghana and Cote d’Ivoire, flowers in Kenya and Ethiopia, improved cassava in Nigeria and rainwater harvesting techniques in Niger. But this notion of “sufficiently productive and profitable” is limited to a geographic, economic and social extent by the heterogeneity that we discuss in the next section.
Below, we summarise some of the overall findings about the main constraints that have been identified and studied in the literature.
Credit, liquidity and savings constraints
Most agricultural technologies come with accompanying costs. If farmers face binding credit constraints and barriers to saving, costs may prevent them from investing in new agricultural technologies. This possibility has motivated a significant amount of research. Much of this work has focused on the adoption of fertiliser and/or improved seeds (which are often complementary inputs). The resulting evidence does not suggest that credit constraints and/or frictions affecting savings can on their own explain the low technology adoption in Africa. Studies on credit and/or savings broadly find increases in adoption, but generally small percentage point increases (though they often amount to large percentage changes relative to the counterfactual). One literature explores factors that may lead to low demand for agricultural credit. Factors include hesitance to list land as collateral, high interest rates, and repayment schedules that do not align well with agricultural cycles (de Janvry and Sadoulet 2020). Abay et al. (2022) show that rainfall variation, which increases production risk, also reduces the demand for credit. Additionally, the low demand for credit is partly reflective of low demand for the inputs themselves (de Janvry and Sadoulet 2020).
Some authors use capital/cash grants to study whether credit constraints matter for farmers. For example, Karlan et al. (2014) gave farmers in Northern Ghana cash grants and did not find significant increases in adoption or yields for those given cash grants. Beaman et al. (2021) find that cash grants increase both adoption and gross profits for farmers who self-select into a group-lending scheme, but that the average returns to farmers who do not select into borrowing are zero.
We would expect input subsidies to increase input demand if credit and/or liquidity constraints prevent farmers from using improved seed and fertilisers. A number of authors study such schemes (see for example Harou et al. 2022, Matsumoto et al. 2013, Dorward and Chirwa 2011, Ricker-Gilbert et al. 2013, and Carter et al. 2021) using a range of methods to identify causal impacts. Jayne et al. (2018) reviewed nearly 80 studies evaluating input subsidy programmes in Africa, concluding that receiving subsidised inputs raises beneficiary households’ grain yields and production levels—at least in the short-term. However, the overall production and welfare effects of subsidy programmes tended to be smaller than expected, for two primary reasons: (1) the subsidy programmes partially crowded out commercial fertiliser demand and hence resulted in less than anticipated increases in total fertiliser use; and (2) the relatively low crop yield response to fertiliser on smallholder-managed fields. Hoffmann and Jones (2021) find that subsidies, combined with training on control of aflatoxin in maize, increase the demand for drying and storage technologies to combat contamination, and that a partial subsidy of a more costly technology is less cost-effective than a full subsidy of a lower-cost technology. Gilligan and Karachiwalla (2023) find that subsidising the full cost of a certified 1kg bag of maize and a 1 litre bottle of herbicide increases the adoption of both inputs. While these papers do find increases in adoption when inputs are subsidised, the estimates vary widely, from quite small to as high as 29 percentage points in Malawi (Carter et al. 2021) - though the subsidy is quite large in this case (a one-time subsidy of US$85, 73% of the cost of fertiliser).
Rather than subsidising technologies directly, some authors have studied subsidising complementary technologies. For example, Aggarwal et al. (2018) study the impacts of providing a limited number of free crop storage devices (PICS bags) and savings accounts on technology use. They find extremely high take up of the PICS bags (see the discussion below on harvest losses) but no change in the use of hybrid seed or fertiliser (though these were already being used at high rates in their sample). Counter to this, Omotilewa et al. (2018) provide farmers in Uganda with free hermetically sealed bags and find an increase in the adoption of hybrid maize that may be more susceptible to pests.
Finally, a set of authors offer farmers credit or savings products directly to study the impacts of these on technology use and yields. Beaman et al. (2023) find strong effects of access to microcredit on input use in Mali. Nakano and Magezi (2020) find small impacts of a loan on fertiliser use amongst those who lacked irrigation, but with no significant improvement in yields in Tanzania. Fink et al. (2020) show that food and cash loans during the lean season in Zambia increase agricultural output and consumption, but via decreases in off farm labour with no impacts on capital inputs or fertiliser use (i.e. the loans do not solve the technology adoption problem). Burke et al. (2019) offer farmers collateralised credit, where the maize harvest is stored by the lender as collateral. This raised farmer revenues, and had an impact on the use of storage technology and the timing of sales. When combined with a savings product, this loan also drove greater farm input investment (Mukherjee et al. 2022).[1]
On the savings side, Duflo et al. (2011) find that limited time vouchers at harvest (addressing commitment problems that prevent farmers from saving for fertiliser purchases) raised adoption of fertiliser by 14 percentage points and Brune et al. (2016) find that a commitment savings programme for inputs (that had 20% take up itself), raised the use of agricultural inputs, but only by 13.3 percentage points. In both cases, the absolute magnitude of use of fertiliser or other inputs remained extremely small. Casaburi and Macchiavello (2019) and Brune et al. (2021) find that dairy farmers in Kenya and agricultural workers in Malawi exhibit strong demand for deferred payments, a saving commitment tool for lumpy expenses, such as animal feeds and agricultural inputs.
Overall, constraints on borrowing and saving seem to affect production decisions by many farmers in Africa, but are not the most binding constraints. Alleviating credit constraints does increase the use of agricultural technologies like improved seed and fertiliser, but ultimately by small overall amounts, not by leaps and bounds.
Insurance constraints
Farmers may be unable to insure risk, which may discourage experimentation and bias their investment decisions towards low-risk, low-return technologies. This bias may be exacerbated if they may care more about downside risk, which may be particularly relevant if they are poor (for example, see Kala 2017).
To better understand the role that risk may play in preventing the adoption of profitable technologies, many researchers have studied the adoption and impacts of rainfall based crop insurance. Largely these studies find (see J-PAL 2016) that the take-up of rainfall insurance at market, or even actuarially fair, rates is very low, likely because existing index insurance products have extremely high basis risk (Carter et al. 2017). Afshar et al. (2021) test two less expensive methods of reducing basis risk - crop simulation models and satellite remote sensing - and find that, compared to crop-cutting estimates (considered best practice), both perform better for the estimation of yield data. Behavioural biases such as loss aversion (Shin et al. 2022) and present bias also appear to limit adoption of these products, which require up-front premium payments for delayed payouts (see Casaburi and Willis 2018 and Serfilippi et al. 2020 for two alternative behavioural explanations for this phenomenon).
In circumstances in which production insurance is provided for free, take up has been extremely high, and with generally strong impacts on the use of agricultural technologies. Kramer et al. (2023) find that the willingness to pay for agricultural insurance in Kenya approximates the commercial price of agricultural insurance. Additionally, farmers prefer payments made to their own mobile money accounts rather than to their spouse or ROSCA, indicating that take up, and thus investments made using the credit, also depends on the design of the insurance scheme. Karlan et al. (2014) find that rainfall insurance provision has positive impacts on the use of fertiliser, increasing use by 23.9 percentage points. Similarly, Elabed and Carter (2018) find that free and subsidised index insurance provided to cotton farmers in Mali significantly increased the area planted to cotton as well as the use of fertiliser and improved seed. However, Ahmed et al. (2020) show that, although take up of (freely provided) rainfall insurance was high in Ethiopia, it had no effects on farming outcomes. Once again, there is substantial heterogeneity. Castaing and Gazeaud (2022) aggregate evidence from six experiments and find that index insurance only increases productive investments by 0.06 to 0.12 SD. Moreover, heterogeneity in effects cannot be explained by basic household characteristics.
Some papers have studied the role of crop price risk instead of weather risk. Karlan et al. (2011) show how an agricultural loan product that had an insurance component partially indemnified farmers against low crop prices and impacted their investment decisions. Farmers offered the insurance spent 17.9 percentage points more on agricultural chemicals (mostly fertiliser) than those who had not been offered the product. Consistent with this, Arouna et al. (2021) find that rice farmers in Benin increase their use of seeds, fertiliser, pesticides, and herbicides - and see resulting increases in yields - when offered a fixed-price contract.
What about the inherent risks of the technology? Adong et al. (2020) study a “risk free sample purchase” sales offer where farmers received a small amount of fertiliser, and only repaid this if their harvest increased more than the cost of fertiliser in Uganda. Farmers who repaid were given the opportunity to place a second order with upfront payment and free delivery. The risk free offer had a take up 73 percentage points higher than a traditional offer, and resulted in a more than 40 percentage point increase in the use of fertiliser.
An important set of technological innovations in agriculture – specifically fertiliser and improved seeds – require upfront investments and generate increased risk for the farmer. Evidence suggests that when this risk can be successfully insured, these risky investments increase, and some of these increases are substantial. However, there remain important challenges in designing low-cost, high quality insurance mechanisms that can serve this role. One option will be to use satellite images, and remote sensing tools to lower these costs, especially as the cost of extremely high resolution satellite images starts to fall and remote sensing-based crop yield measurement improves (Benami et al. 2021, Lobell et al. 2020). The use of geo-referenced photographs of crop losses also holds promise (Ceballos et al. 2019) and is currently being tested in Kenya (Kramer 2023). On the contractual side, Carter (2022) shows that the sort of loss indexes used for insurance contracts can also be used to trigger lines of credit and as the basis for variable term or commitment savings accounts. Because of the problem of imperfect determination of losses when using remote sensing tools (see the Benami et al. 2021 discussion of “basis risk”), these alternative financial instruments may promise a safer and lower cost agricultural risk management solution for small-scale farmers.
Knowledge constraints
One possible explanation for the low adoption of technologies is that farmers either do not know about new technologies, or they do not have the relevant information about the returns to the technology and how to use it effectively. The literature in this area is vast and includes not just understanding whether providing farmers with information helps, but how best to provide this information, and the role of learning in social networks. A wide variety of studies evaluate extension programmes, public and private. Again, a full review of those is beyond the scope of this piece. But, largely, most of these studies do not find transformational effects on technology adoption (see Caldwell et al. 2019, and Bridle et al. 2019, for good overviews).
In terms of some more recent examples of studies in Africa, Fabregas et al. (2017) evaluate the effectiveness of two different extension approaches on farmers’ knowledge, beliefs and input choices: (i) farmers attend a farmer field day with demonstration plots, and (ii) farmers receive agricultural messages on their mobile phone. The field days increased knowledge but had small effects on input use (at most 4 percentage point increases), the messages did not change input use. Similarly, Kondylis et al. (2017) study a training programme that resulted in small increases in adoption (of 6 percentage points for input use, by 15 percentage points for pit planting and tillage), with no diffusion to other farmers. Bonan et al. (2023) study an extension programme that provided marketing and technology information to farmers in Uganda about new oilseed crops. They show the information provision led farmers to revise their expectations about the yields and prices of these crops. As a result, the adoption of the new oilseed crops increased by 15%. Bernard et al. (2017) show that information on quality increased switching to higher-quality fertiliser by about 25 percentage points. Kondylis et al. (2017) show that training contact farmers who then would train others in Mozambique raises adoption of the technology by the contact farmers themselves, but unfortunately not by other farmers. Horner et al. (2019) find that an agricultural training programme with video messaging increased fertiliser use by 4 percentage points, lime use by 24 percentage points and use of improved seed by 6 percentage points. Maertens et al. (2021) find that farmer demonstration plots had a significant effect on knowledge dissemination and on adoption of planting and management practices, but that farmer farmer field days had no such effect in Malawi. Aker and Jack (2023) find that training on rainwater harvesting techniques significantly increased the extensive and intensive margins of adoption (an increase of over 90 percentage points), and also show that there are spillovers from adoption. The training was similarly effective at increasing adoption when combined with other financial incentives, namely unconditional and conditional cash transfers.
Learning by doing may be more effective than training programmes. “Smart subsidies” that are temporary in nature can encourage experimentation with new technologies, driving increases in usage that persist even once the subsidies are removed, as Carter et al. (2021) find among maize farmers in Mozambique. However, learning by doing may be slowed by inference problems due to heterogeneous returns.
They may also be slowed when the learning is about technologies that only infrequently reveal their benefits, such as stress tolerant seeds or insurance. The Boucher et al. (forthcoming) study of a bundle of drought tolerant seeds with index insurance finds that farmers who experienced drought conditions and other yield losses intensified their use of these technologies the year following losses. Predictably, perhaps, those who did not experience the losses walked away from the technology in the next year. While subsidies can indeed encourage experimentation with new technologies (Carter et al. 2021), making those subsidies “smart” for technologies that infrequently reveal their benefits is a rather more difficult problem that would seem to merit further investigation.
Learning by doing can also be impeded by the presence, or beliefs about the presence, of low quality or inauthentic technologies in the market (Michelson et al. 2021, Ashour et al. 2019, Bold et al. 2017). Hoel et al. (2024) caution that learning about quality can be difficult or near impossible if there are incorrect (misattribution of bad luck to poor quality inputs) or uncertain beliefs (ambiguity) about product quality. Looking in particular at how farmers learn, a number of studies document the role of social networks. Pineapple farmers in Ghana use more inputs when their neighbours are surprisingly successful in previous periods (Conley and Udry 2010). Carter et al. (2021) find evidence of increased fertiliser adoption by those in the social network of subsidised farmers. In a framed field experiment in Ghana, Ambler et al. (2023) find that farmers who are provided with a subsidy adopt conservation agriculture in the short and long term, as do those provided with information on long term returns, but not other types of information. Beaman et al. (2021) show that adoption is higher if the intervention targets who to tell about a new technology based on who is central in the social networks in the village. This raises adoption of pit planting, a very specific technology, in Malawi by 30%. Abdulai (2023) documents complementarities between social networks and improved extension services in the adoption of a new wheat variety in Ethiopia. Tjernström (2017) studies the interaction of the usefulness of social networks with variations in soil quality in the village. She finds important social network effects but that these network effects are weaker in villages in which soil quality is more varied, which illustrates how heterogeneity in returns can handicap network effects.We return to heterogeneity in more detail in the next section.
A more nascent literature examines how digitally provided information can increase take-up of agricultural technologies. Van Campenhout et al. (2020) find that farmers in Uganda who watched a video about input use and improved maize practices performed significantly better on a knowledge test than those who did not watch the video, were more likely to use fertiliser and had yields that were 10.5% higher. The addition of IVR messaging, nor SMS messaging, had any impact on these outcomes. Mawunyo Dzanku and Osei (2023) find that voice messages sent to farmers in Mali led to increased adoption of agricultural inputs, the magnitude was not large enough to spur improvements in output or profits.
Fertiliser, improved seed, irrigation, financial products to improve access to credit and insurance, and marketing structures are widely thought to complement one another. Indeed, most hybrid seeds are expressly bred to respond well to inorganic fertiliser application. An enduring puzzle, therefore, is why even among households that do adopt improved inputs, they are often not used synergistically, on the same crop and plot. Sheahan and Barrett (2017) find in LSMS-ISA plot-level data from Ethiopia and Niger, for example, that joint use of multiple fertiliser, improved seed or irrigation inputs occurs on only 13-14% of plots that use at least one of those inputs. This seems to reflect a knowledge gap that might be addressed through extension services. But Africa’s extension services are themselves largely in disarray (Jayne et al. 2023).
Overall, it seems that extension and information programmes have limited effects on adoption, except perhaps for the case where the technologies or the crops are truly new. Social networks do matter a lot, especially when thinking about who to target, though many effective interventions are extremely costly to scale and replicate in policy. While most of the literature focuses on farmers’ acquisition of technical knowledge, Laajaj and Macours (2021) show that, in a sample of Kenyan farmers, cognitive and noncognitive skills are correlated with yields in a sample of Kenyan farmers.
Limited market access
Lack of market access may deter farmers from adopting new agricultural technologies by increasing the cost of inputs and decreasing the gain from selling outputs. Farmers across the continent face high transaction costs across multiple dimensions: high transportation costs; poor supply chain investments; market power among sellers of inputs and buyers of outputs; and imperfect information about price and quality.
The clearest barriers to market access arise from high transportation costs and poor infrastructure. Atkin and Donaldson (2015) find that the effect of distance on prices of traded goods in Nigeria and Ethiopia is four or five times the effect in the United States. This is consistent with the fact that direct trucking costs in Africa are much larger than those in developed countries (Teravaninthorn and Raballand 2009). Median trade costs in Africa are about five times higher than everywhere else in the world (Porteous 2019).
These costs are likely to deter technology adoption, although there is yet a lot to learn. For example, Aggarwal et al. (2022) study the effects of poor market access in Tanzania on input adoption. They find that doubling transport costs is associated with a reduction in the adoption of fertilisers by 14 percentage points (25%), while reducing travel costs by 50% doubles their adoption (we discuss fertiliser pricing in more detail in section 4). Casaburi et al. (2013) study the impacts of road improvements on agricultural markets in Sierra Leone and find that markets (and hence prices) are better integrated in markets that are closer to improved infrastructure. Dillon and Tommaselli (2022) and Aggarwal et al. (2023) show that input fairs can increase input adoption.
Transaction costs and poor infrastructure can also prevent optimal timing of market access. Improved storage bags have been shown to reduce post-harvest losses and allow optimal timing of crop sales (Aggarwal et al. 2018 and Omotilewa et al. 2018). Burke et al. (2019) show that harvest-time loans can enable providing a vehicle for maize farmers in Kenya to store their crops and sell at times of peak prices. Other recent studies focusing on combinations of credit and storage technologies to delay maize sales include Channa et al. (2022) and Le Cotty et al. (2019). Delavalladde and Godlonton (2023) show that a warrantage in Burkina Faso led farmers to store more grains and pulses, and obtain higher prices by selling over an extended period of time. This idea was also studied by Casaburi et al. (2014) in Sierra Leone for palm oil. Cardell and Michelson (2022) however have shown using 20 years of data from 787 markets in 20 Sub-Saharan African countries that prices fail to rise after harvest approximately 28% of the time and argue that farmers storing to sell later in the year face what is known as “flat price risk”.
Farmers’ market access is typically mediated by intermediaries on both the input and the output side. Evidence on the extent to which these intermediaries increase costs or reduce market integration is mixed (Dillon and Dambro 2017). Some studies find that traders exercise a great deal of market power over farmers’ access to output markets (see Bergquist and Dinerstein 2020, Casaburi et al. 2013 and Newman et al. 2018). An older literature based on analyses of traders’ margins and cross-market price variation (reviewed in Dillon and Dambro 2017) often finds evidence of relatively robust competition in output markets, as do Casaburi and Reed (2022) based on responses to world price shocks and to a randomised subsidy to cocoa traders in Sierra Leone. It seems likely that the mixed evidence reflects a great deal of heterogeneity in the reality on the ground. Less evidence exists regarding the functioning of intermediaries on the input side of the market and few research studies have focused on agri-dealers.
Finally, markets in the region are often not well integrated, either spatially or temporally, in part due to the challenges of gathering information about prices or the availability of buyers and sellers in distant places. This is slowly changing with the growth in cell phone adoption and use, even in Africa (for example, see Aker and Mbiti 2010). Interventions that directly send price information to smallholder farmers via mobile phones have had mixed results (Nakasone et al. 2014, Hildebrant et al. 2023). However, search technologies may be more usefully employed by intermediaries, who operate at the scale required to conduct cross-market trade. Bergquist et al. (2022) find increases in trade flows and reductions in price dispersion from trader take-up of a mobile agricultural marketplace in Ugandan output markets. Although farmers rarely use the mobile marketplace directly, those in surplus areas, where market prices are driven up as a result of the intervention, see increases in revenues. Wiseman (2023) finds a mobile phone application that provides price information and official border costs to traders in Kenya alters traders’ choices of markets and border crossings, and affects local market prices. Barrett et al. (2020) provide a useful review of recent work documenting innovations in agricultural value chains linking farmers to food consumers. Institutions such as commodity exchanges likely also have a role to play in reducing frictions in matching supply to demand. The small amount of evidence available on these to-date comes from Ethiopia and Ghana (Nyarko and Pellegrina 2022).
Overall, a lack of market access implies that farmers cannot easily sell their output or easily access inputs, and that the profits from technology use may be low given the current state of infrastructure. There is still much more work to be done in better understanding the role of input and output markets for overall agricultural productivity in Africa. Infrastructure may also interact in important ways with other constraints, as illustrated by some of these studies.
Market for quality
A set of authors has specifically studied the lack of markets for quality, i.e. the lack of different prices for varying quality of crops, in Africa. In theory, adoption of some technologies or techniques may enable farmers to upgrade the quality of their output and earn higher prices and margins. However, if quality price premia are not available to farmers due to challenges with quality measurement, contract enforcement, appropriate storage or transport to maintain quality, or low access to markets with high consumer willingness to pay for quality, then adoption of such technologies will fail to yield benefits.
For example, Prieto et al. (2021) find that Senegalese traders and consumers have both a willingness to pay for drier maize that is safer to consume and some ability to assess moisture content, but that consumers in particular have low confidence in their own ability to assess quality.
There is evidence that farmers have the ability to upgrade quality when given reason to do so, in at least some settings. However, it is less clear whether they are able to do so in response to appropriate price incentives alone, or whether additional knowledge or access to inputs is required. Bernard et al. (2017) study the market for quality of onions in Senegal and find that informing farmers about an upcoming change to the way quality would be assessed in markets raises adoption of quality enhancing inputs by 27 percentage points. Magnan et al. (2021) study whether a price premium for groundnuts in Ghana that comply with aflatoxin regulations changes adoption of the technologies that help deal with aflatoxin. They find that a price premium increases the adoption of recommended post-harvest practices more than training on aflatoxin prevention alone, and has a similar impact on aflatoxin contamination as provision of drying equipment. Macchiavello and Miquel-Florensa (2019) find that combining extension and training with a guarantee that a certain fraction of a quality price premium would be paid to farmers (rather than captured by intermediaries), led to substantial quality upgrading of coffee in Colombia, although it is not possible to separate out the role played by different components of the programme. Hoffmann et al. (2023) find that a modest quality premium more than doubled semi-subsistence farmers’ adoption of a safety-enhancing input, and that this effect was driven by farmers who were motivated by a combination of health benefits and financial rewards.
One important question is whether farmers do, in fact, face quality price premia, and if not, why not. Bold et al. (2022) find maize farmers in Uganda are able to upgrade quality when offered premium prices for doing so, but that the market offers roughly zero return to quality, though a previous study finds a significant price penalty at farmgate for very low quality (Kadjo et al. 2016). Several studies find returns to observable quality at the retail and wholesale level in informally marketed agricultural produce (Kadjo et al. 2016, Prieto et al. 2021, Hoffmann et al. 2022), and to unobservable food safety in formally processed, branded products, where reputation and regulatory enforcement may play a role (Hoffmann et al 2020). Casaburi and Reed (2022) find that offering quality incentives to cocoa traders in Sierra Leone does improve the quality they supply, although this likely reflects substitution across traders rather than an aggregate increase in the quality supplied by the market as a whole. They find that traders do pass on some of these returns to farmers in the form of more in-kind credit. Macchiavello and Morjaria (2021) investigate the role of competition among coffee mills in Rwanda, and find that an increase in competition between buyers (in an environment with a fairly high average level of competition) actually decreases the quantity and quality of coffee supplied, likely because it undermines relational agreements through which mills provide farmers with access to inputs and loans pre-harvest.
Why quality premia do not exist more often in the absence of experimental interventions is an open question. Hoffmann et al. (2020) suggest that this may in part reflect a lack of both awareness and willingness to pay for third-party verification of food safety on the part of African consumers, in the context of aflatoxin-safe maize flour in Kenya.
Inability to accurately assess quality may be another contributing factor on both the input and the output side, but evidence is mixed. Michelson et al. (2023) review the evidence regarding the quality of non-labour agricultural inputs in Sub-Saharan Africa and show that research has mostly focused on urea fertiliser and glyphosate herbicide. There are disputes over the quality of fertiliser in different countries; Bold et al. (2017) argue that there is significant adulteration of urea fertilisers and herbicides, respectively, in Uganda, but Sanabria et al. (2013), Sanabria et al. (2018a), Sanabria et al. (2018b), Ashour et al. (2019), Hoel et al. (2024), and Michelson et al. (2021) provide evidence that urea fertiliser is of good quality across multiple countries.
While urea fertiliser quality has been shown to be good, glyphosate quality is more variable; insufficient evidence exists regarding other fertilisers, pesticides, and seed. Evidence suggests that farmers believe input quality is low (Ashour et al. 2019, Bold et al. 2017), and that while these beliefs do not always reflect market realities, they inform farmer investment, willingness to pay, and expected returns (Michelson et al. 2021, Gharib et al. 2021, Maertens et al. 2022). Some evidence suggests that farmer uncertainty about input quality may reduce complementary investment in other inputs. Bulte et al. (2014) experimentally vary farmer information about both the quality of the maize seed they are provided (hybrid or open pollinated variety) and farmer uncertainty about the quality type and show that farmers who have more uncertainty about the seed quality reduce labour investment and report relatively lower maize production and yields. Even so, beliefs about input quality respond to information; Michelson et al. (2024) randomise an information campaign about fertiliser quality in Tanzania and find that farmer fertiliser purchases and use increase among farmers in treated marketsheds.
While there is evidence that low-cost changes such as the use of control samples can significantly improve the performance of food safety laboratories (Herrman et al. 2020), the scale, cost, and impact of investments that would be required to meaningfully alter quality assurance capacity and change related incentives is an open question. Digital tools can also play a role - Miehe et al. (2023) test an information clearing house akin to yelp.com and find that exposed farmers are more likely to take up hybrid maize seeds and experience higher levels of productivity. The intervention also resulted in higher trust in certain agro-dealers. Where input quality is low, quality verification schemes can encourage farmers to switch input suppliers, resulting in higher yields (Hsu and Wambugu 2023).
Quality verification can also affect market outcomes. Gilligan et al. (2022) evaluate a government-run certification scheme for hybrid maize, inorganic fertiliser, and glyphosate herbicide in Uganda. Providing information about the new verification scheme to farmers and subsidies for verified products in a subset of markets improved the average quality (and prices) of fertiliser in the treated markets and led to increased adoption of the inputs.
On the output side, Prieto et al. (2021) find that Senegalese traders and consumers have both a willingness to pay for drier maize that is safer to consume and some ability to assess moisture content, but that consumers in particular have low confidence in their own ability to assess quality.
Imperfect labour markets
Only a handful of studies have examined the role that poorly functioning labour markets play in constraining agricultural productivity in Africa, or how best to improve the functioning of these labour markets. One hypothesis for the low use of improved technology and hence low agricultural productivity is that, given the low population density in many African economies and the highly seasonal and often time-sensitive demands for labour in agriculture, labour markets are extremely constrained. Farmers may find it hard to hire or provide the right types of labour at the right times, imposing constraints on their production. Using World Bank LSMS data from five countries, Dillon and Barrett (2017) finds strong evidence of separation failures in agricultural production. This may be partially driven by liquidity constraints (Fink et al. 2020). Jones et al. (2022) show a link between poorly functioning labour markets and limited farm investment, in the context of irrigation investments in Rwanda while Caunedo and Kala (2021) find similar results for mechanisation in India. Carranza et al. (2022) shows that sharing norms may discourage labour supply in the agricultural processing sector in Ivory Coast. There is very little literature here, partly because it is hard to design interventions in labour markets that we think may improve labour allocation and be implementable from a policy perspective.
Imperfect land markets
While there is widespread recognition that land markets are imperfect in sub-Saharan Africa, there has been little work on understanding the ways to improve their functioning and efficiency. Imperfect land markets and poorly defined property rights for farmers may explain part of the low level of investment in agricultural technologies as farmers may not feel confident in their access to the future returns on these investments. For example, Goldstein and Udry (2008) find that the insecurity of property rights is responsible for suboptimal fallowing length, resulting in significantly lower production of crops. A small literature documents the impacts of improvements in property rights on the use of technology, but we still have a lot to learn. Agyei-Holmes et al. (2020) study a pilot land registration programme in Ghana and find that it, in fact, does not affect agricultural investments, but rather releases labour for higher return off-farm uses. Ali et al. (2014) look at a land tenure programme in Rwanda and find the opposite— they find large effects on agricultural investment and on the use of soil conservation practices, especially for female-headed households. Similarly, Wren-Lewis et al. (2020) find that land certification in Benin led to less forest loss, partly because farmers invested more in their existing land, lowering the need to clear land. Finally, Goldstein et al. (2018) study the same certification programme in Benin and find that the programme increased investment, especially by women, who had low tenure rights to begin with. After the programme, women were more likely to leave land fallow, a soil fertility investment.
Despite land abundance in some areas of the continent, land rental has become quite important in some places. Burchardi et al. (2019) cites national census statistics from Uganda that 38% of crop-producing households rent land through sharecropping arrangements. Less formal statistics indicate that 20-25% of Ethiopian farms similarly sharecrop land. As has long been appreciated in economics, the Marshallian disincentives of sharecropping potentially dampen agricultural productivity, despite the advantages sharecropping brings in the form of minimal capital requirements and partial insurance. In an experimental study, Burchardi et al. (2019) show that moving Uganda farmers toward fixed rent contracts resulted in a remarkable 60% productivity increase. Finding ways to create the credit and insurance institutions that would allow a shift to fixed rent contracts would thus seem to be an important, if yet underexplored, way to boost agricultural productivity.
The importance of rural land markets is growing in many countries of Sub-Saharan Africa (Deininger et al. 2017, Jayne et al. 2021). While large-scale transactions receive the largest attention in policy debates, most transactions occur among small or medium farmers (Jayne et al. 2016). Nevertheless, a growing of body of work suggests that land market imperfections inhibit the growth of particularly productive farms (Restuccia and Santaeulàlia-Llopis 2017); preclude farmers from capturing potential returns to scale associated with mechanisation (Foster and Rosenzweig 2022); and slow the development of robust value chains that can support technological innovation (Barrett et al. 2020). Market imperfections in rural land markets can include social norms; in Malawi, Krah et al. (2024) show that field characteristics including soil quality explain less than a third of land rental rates and find that villages with stronger experimentally-elicited fairness norms have tighter ranges in rental rates. Rental rates are likely to be capped in villages with stronger fairness norms and may serve to protect tenants from rent increases.
Acampora et al. (2022) use an RCT in Western Kenya to subsidise land rentals and find that this improves equity and increases output and value added on the rented plot. They highlight how land market imperfections matter and how these interact with labour market frictions. Customary land tenure systems inhibit the commercialisation of land, but they provide guarantees of some access to land for most people in rural Africa and play a central role in informal social protection. Transformation of land tenure systems to facilitate agricultural innovation requires attention to these broader consequences for the distribution of power and welfare in rural communities.
Perhaps because of these limitations in the land tenure system, a number of countries (particularly Ethiopia, Nigeria, Mozambique and Sudan) began facilitating large-scale land acquisitions by outside investors. Deininger et al. (2011) calculate that as many as 40 million hectares had been transferred under these schemes, with a median project size in the thousands of hectares. While often controversial, these programmes were justified in terms of the productivity gains imagined to occur directly through large-scale farming, and with the spillovers from the large-scale to smaller-scale farms (Collier and Venables 2012). As summarised by Deininger and Goyal (2023), there is at best modest evidence that these large-scale land transfers have succeeded in generating productivity gains and spillovers.
It is worth noting that properly functioning rental markets for agricultural equipment could enable smaller farms to overcome scale-related barriers. However, barriers in the rental market often preclude this (Dillon and Barrett 2017, Sheahan and Barrett 2017, Caunedo and Kala 2021, Daum 2021). There is a need for more research in this space.
Externalities, land and climate change
A large and growing literature documents the negative impacts of climate change on agricultural productivity across the world, especially in Africa (Ortiz-Bobea et al. 2021, Barrett et al. 2023). We do not review that literature here given our focus on agricultural technologies in Africa. Instead, we focus on the diffusion of technologies and strategies that help farmers deal with these impacts of climate change.
A significant portion of African farmland has one or more of the following conditions that reduce crop response to fertilisers and other inputs: acidic soils, low soil organic carbon, sandy soils, and micronutrient deficiencies. Until these soil issues are somehow addressed, input intensification may be limited (Sanchez 2019). However, the adoption of a wide variety of natural resource management practices remains very low in Africa (see Stevenson and Vlek (2018) for case studies on Zimbabwe, Zambia, Malawi, Niger, Mozambique, Kenya and Rwanda). Much of the existing work tries to incentivise adoption of these practices in a variety of ways. For example, Jayachandran et al. (2017) provide incentives to forest-owning households to conserve their forests in Uganda and find that these incentives do reduce deforestation without creating additional deforestation elsewhere. Oliva et al. (2020) study subsidies for the take up of agroforestry (trees that improve soil fertility over the long term) in Zambia. Much as the subsidies do increase take up, the follow through to future investments in the trees is low and uncorrelated with the initial subsidy (the follow through seems to require additional incentives). Coppock et al. (2022) study the effects of a community-based rangeland and cattle management programme in Namibia, which was successful in improving community resource management but had no effects on cattle productivity or incomes. Aker and Jack (2023) find training farmers in Niger on an agricultural technique that helps reduce land degradation and restore soil fertility increased adoption by over 90 percentage points, while adding conditional or unconditional cash transfers had no additional effect. Jagnani et al. (2021) find that Kenyan maize farmers respond to changes in pest ecology induced by rising temperatures by increasing pesticide use, which necessitates reducing fertiliser application given binding budget constraints.
References
Abay, K A, B Koru, J Chamberlin, and G Berhane (2022), “Does rainfall variability explain low uptake of agricultural credit? Evidence from Ethiopia”, European Review of Agricultural Economics, 49(1): 182-207.
Abdulai, A (2023), “Information Acquisition and the Adoption of Improved Crop Varieties”, American Journal of Agricultural Economics, 105(4): 1049-1062.
Acampora, M, L Casaburi, and J Willis (2022), “Land Rental Markets: Experimental Evidence from Kenya.”, STEG Working Paper.
Adong, A, J Tinker, D Levine, S Mbowa, and T Odokonyero (2020), “Encouraging Fertilizer Adoption through Risk Free Sales Offer: A Randomized Control Trial in Uganda”, World Development Perspectives, 19: 100230.
Afshar, H M, T Foster, T P Higginbottom, B Parkes, K Hufkens, S Mansabdar, F Ceballos and B Kramer (2021), “Overcoming Basis Risk in Agricultural Index Insurance using Crop Simulation Modeling and Satellite Crop Phenology”, EGU General Assembly.
Aggarwal, S, B Giera, D Jeong, J Robinson, and A Spearot (2022), “Market Access, Trade Costs, and Technology Adoption: Evidence from Northern Tanzania”, Review of Economics and Statistics, forthcoming.
Aggarwal, S, D Jeong, N Kumar, D S Park, J Robinson, and A Spearot (2023), "Shortening the Path to Productive Investment: Evidence from Input Fairs and Cash Transfers in Malawi."
Aggarwal, S, E Francis, and J Robinson (2018), “Grain Today, Gain Tomorrow: Evidence from a Storage Experiment with Savings Clubs in Kenya”, Journal of Development Economics, 134: 1-15.
Agyei-Holmes, A, N Buehren, M Goldstein, R D Osei, I Osei-Akoto, and C Udry (2020), “The Effects of Land Title Registration on Tenure Security, Investment and the Allocation of Productive Resources”, Global Poverty Research Lab Working Paper No. 20-107.
Ahmed, S, C McIntosh, and A Sarris (2020), “The Impact of Commercial Rainfall Index Insurance: Experimental Evidence from Ethiopia”, American Journal of Agricultural Economics, 102(4): 1154-1176.
Aker, J C and B K Jack (2023), “Harvesting the Rain: The Adoption of Environmental Technologies in the Sahel”, Review of Economics and Statistics, forthcoming.
Aker, J C and I M Mbiti (2010), “Mobile Phones and Economic Development in Africa”, Journal of Economic Perspectives, 24(3): 207-32.
Ali, D A, K Deininger and M Goldstein (2014), “Environmental and Gender Impacts of Land Tenure Regularization in Africa: Pilot Evidence from Rwanda”, Journal of Development Economics, 110: 262-275.
Ambler, K, A de Brauw and M Murphy (2023), “Increasing the adoption of conservation agriculture: A framed field experiment in northern Ghana”, Agricultural Economics, 54(5): 742-756.
Appiah, E, K Baldwin, D S Karlan and C Udry (2020), "How Political Insiders Lose Out When International Aid Underperforms: Evidence from a Participatory Development Experiment in Ghana." Unpublished.
Arouna, A, J D Michler and J C Lokossou (2021), "Contract Farming and Rural Transformation: Evidence from a Field Experiment in Benin", Journal of Development Economics, 151: 102626.
Ashour, M, D O Gilligan, J B Hoel and N I Karachiwalla (2019), "Do beliefs about herbicide quality correspond with actual quality in local markets? Evidence from Uganda", The Journal of Development Studies, 55(6): 1285-1306.
Ashraf, N, X Giné and D Karlan (2009), "Finding Missing Markets (and a Disturbing Epilogue): Evidence from an Export Crop Adoption and Marketing Intervention in Kenya", American Journal of Agricultural Economics, 91(4): 973-990.
Atkin, D and D Donaldson (2015), "Who's Getting Globalized? The Size and Implications of Intra-national Trade Costs", NBER Working Paper No. 21439.
Barrett, C B, A Ortiz-Bobea and T Pham (2023), “Structural Transformation, Agriculture, Climate, and the Environment”, Review of Environmental Economics and Policy, 17(2): 195-216.
Barrett, C B, T Benton, J Fanzo, M Herrero, R J Nelson, E Bageant, ... and S Wood (2022), “Socio-technical innovation bundles for agri-food systems transformation.” Springer Nature.
Barrett, C B, T Reardon, J Swinnen, and D Zilberman (2022), "Agri-food Value Chain Revolutions in Low-and Middle-Income Countries", Journal of Economic Literature, 60(4): 1316-1377.
Beaman, L, A BenYishay, J Magruder, and A M Mobarak, (2021), “Can Network Theory-based Targeting Increase Technology Adoption?”, American Economic Review, 111(6): 1918-43.
Beaman, L, D Karlan, B Thuysbaert, and C Udry (2023), “Self-selection into Credit Markets: Evidence from Agriculture in Mali”, Econometrica, 91(5).
Benami, E, Z Jin, M R Carter, A Ghosh, R J Hijmans, A Hobbs, B Kenduiywo and D B Lobell (2021), "Uniting Remote Sensing, Crop Modelling and Economics for Agricultural Risk Management.", Nature Reviews Earth & Environment, 2(2): 140-159.
Bergquist, L F and M Dinerstein (2020), "Competition and Entry in Agricultural Markets: Experimental Evidence from Kenya", American Economic Review, 110(12): 3705-47.
Bergquist, L F, C McIntosh and M Startz (2022), “Search Costs, Intermediation, and Trade: Experimental Evidence from Ugandan Agricultural Markets”, Working Paper.
Bernard, T, A De Janvry, S Mbaye and E Sadoulet (2017), "Expected Product Market Reforms and Technology Adoption by Senegalese Onion Producers", American Journal of Agricultural Economics, 99(4): 1096-1115.
Binswanger-Mkhize, H, and A F McCalla (2010), "The Changing Context and Prospects for Agricultural and Rural Development in Africa", Handbook of Agricultural Economics, 4: 3571-3712.
Bold, T, K C Kaizzi, J Svensson and D Yanagizawa-Drott (2017), "Lemon Technologies and Adoption: Measurement, Theory and Evidence from Agricultural Markets in Uganda", The Quarterly Journal of Economics, 132(3): 1055-1100.
Bold, T, S Ghisolfi, F Nsonzi and J Svensson (2022), "Market Access and Quality Upgrading: Evidence from Four Field Experiments", American Economic Review, 112(8), 2518-2552.
Bonan, J, H Kazianga and M Mendola (2023), “Agricultural Transformation and Farmers' Expectations: Experimental Evidence from Uganda.” Working Paper.
Bossuroy, T, M Goldstein, D Karlan, H Kazianga, W Pariente, P Premand, C Thomas, C Udry, J Vaillant, and K Wright (2022), "Pathways out of Extreme Poverty: Tackling Psychosocial and Capital Constraints with a Multi-faceted Social Protection Program in Niger", Nature, 605: 291–297.
Boucher, S, M R Carter, T Lybbert, J Malacarne, P Marenya and L Paul (forthcoming). “Bundling Genetic and Financial Technologies for More Resilient and Productive Small-scale Agriculture,” Economic Journal.
Bridle, L, J Magruder, C McIntosh and T Suri (2019), “Experimental Insights on the Constraints to Agricultural Technology Adoption”, Working Paper. Agricultural Technology Adoption Initiative, J-PAL (MIT) and CEGA (UC Berkeley).
Brune, L, E Chyn and J Kerwin (2021), "Pay me later: Savings constraints and the demand for deferred payments." American Economic Review, 111(7): 2179-2212.
Brune, L, X Giné, J Goldberg, and D Yang (2016), “Facilitating Savings for Agriculture: Field Experimental Evidence from Malawi”, Economic Development and Cultural Change, 64(2): 187-220.
Bulte, E, G Beekman, S Di Falco, J Hella, and P Lei (2014), “Behavioral responses and the impact of new agricultural technologies: Evidence from a double‐blind field experiment in Tanzania.” American Journal of Agricultural Economics, 96(3): 813-830.
Burchardi, K, S Gulesci, B Lerva and M Sulaiman (2019), “Moral Hazard: Experimental Evidence from Tenancy Contracts”, Quarterly Journal of Economics, 134(1): 281–347.
Burke, M, L F Bergquist, and E Miguel (2019), “Sell Low and Buy High: Arbitrage and Local Price Effects in Kenyan Markets”, The Quarterly Journal of Economics, 134(2): 785-842.
Caldwell, R, R Lambert, J Magruder, C McIntosh, and T Suri (2019), "Improving Agricultural Extension and Information Services in the Developing World", VoxDev, Accessed 2 Jun. 2021.
Cardell, L and H Michelson (2022), “Price Risk and Staple Grain Storage Decisions: New Insights into a Long-Standing Puzzle”, Working Paper.
Carranza, E, A Donald, F Grosset and S Kaur (2022), “The Social Tax: Redistributive Pressure and Labor Supply” NBER Working Paper No. w30438.
Carter, M (2022), “Can digitally‐enabled financial instruments secure an inclusive agricultural transformation?”, Agricultural Economics.
Carter, M, A De Janvry, E Sadoulet and A Sarris (2017), "Index insurance for developing country agriculture: a reassessment", Annual Review of Resource Economics, 9: 421-438.
Carter, M, R Laajaj, and D Yang (2021), “Subsidies and the African Green Revolution: direct effects and social network spillovers of randomized input subsidies in Mozambique.” American Economic Journal: Applied Economics, 13(2): 206-229.
Casaburi, L and J Willis (2018), “Time versus State in Insurance: Experimental Evidence from Contract Farming in Kenya”, American Economic Review, 108(12): 3778-3813.
Casaburi, L and R Macchiavello (2019), "Demand and supply of infrequent payments as a commitment device: evidence from Kenya." American Economic Review, 109(2): 523-555.
Casaburi, L, R Glennerster and T Suri (2013), “Rural Roads and Intermediated Trade: Regression Discontinuity Evidence from Sierra Leone”, Available at SSRN 2161643.
Casaburi, L, R Glennerster, and T Suri, and S Kamara (2014), “Providing collateral and improving product market access for smallholder farmers. A randomised evaluation of inventory credit in Sierra Leone”, 3ie Impact Evaluation Report 14.
Casaburi, L and T Reed (2022), “Using individual-level randomized treatment to learn about market structure”, American Economic Journal: Applied Economics, 14(4): 58-90.
Castaing, P, and J Gazeaud (2022), "Do Index Insurance Programs Live Up to Their Promises? Aggregating Evidence from Multiple Experiments”, World Bank Policy Research Working Paper 10161.
Caunedo, J, and N Kala (2021), “Mechanizing Agriculture: Impacts on Labor and Productivity”, Dispersion in Financing Costs and Development.
Ceballos, F, B Kramer and M Robles (2019), “The feasibility of picture-based insurance (PBI): Smartphone pictures for affordable crop insurance.” Development Engineering, 4: 100042.
Chambers, R (2014), Rural development: Putting the last first. New York: Routledge.
Channa, H, J Ricker-Gilbert, S Feleke and T Abdoulaye (2022), “Overcoming smallholder farmers’ post-harvest constraints through harvest loans and storage technology: Insights from a randomized controlled trial in Tanzania”, Journal of Development Economics, 157: 102851.
Collier, P and A J Venables (2012), "Land Deals in Africa: Pioneers and Speculators." Journal of Globalization and Development, 3(1).
Conley, T G and C R Udry (2010), “Learning about a new Technology: Pineapple in Ghana”, American Economic Review, 100(1): 35-69.
Coppock, D L, L Crowley, S L Durham, D Groves, J C Jamison, D Karlan, B E Norton, and R D Ramsey (2022), “Community-based rangeland management in Namibia improves resource governance but not environmental and economic outcomes”, Communications Earth & Environment, 3(1): 32.
Crepon, B, F Devoto, E Duflo, and W Parienté (2015), “Estimating the impact of microcredit on those who take it up: Evidence from a randomized experiment in Morocco”, American Economic Journal: Applied Economics, 7(1): 123-50.
Daum, T (2021), “Farm robots: ecological utopia or dystopia?”, Trends in Ecology & Evolution, 36(9): 774-777.
de Janvry, A and E Sadoulet (2020), “The puzzle of lagging Sub-Saharan Africa agriculture: Toward a theory of connectedness”, Working Paper.
de Janvry, A, E Sadoulet and T Suri (2017), "Field experiments in developing country agriculture." In Handbook of Economic Field Experiments, vol. 2, pp. 427-466. North-Holland.
Deininger, K, D Byerlee, J Lindsay, A Norton, H Selod and M Stickler (2011), “Rising global interest in farmland: Can it yield sustainable and equitable benefits?”, Washington, DC: World Bank.
Deininger, K, S Savastano and F Xia (2017), "Smallholders’ land access in Sub-Saharan Africa: A new landscape?", Food Policy, 67: 78-92.
Deininger, K and A Goyal (2023), “Land policies and institutions for equitable and resilient growth in Africa,” World Bank Working Paper.
Deutschmann, J W, M Duru, K Siegal and E Tjernström (2019), “Can Smallholder Extension Transform African Agriculture?”, NBER Working Paper 6054.
Deutschmann, J W, T Bernard and O Yameogo (2021), “Contracting and quality upgrading: evidence from an experiment in Senegal”, Working Paper.
Dillon, B, and C B Barrett (2017), "Agricultural factor markets in Sub-Saharan Africa: An updated view with formal tests for market failure." Food Policy, 67: 64-77.
Dillon, B, and C Dambro (2017), “How competitive are crop markets in sub‐Saharan Africa?”, American Journal of Agricultural Economics, 99(5): 1344-1361.
Dillon, A and N Tomaselli (2022), "Making Markets: Experiments in Agricultural Input Market Formation."
Dorward, A and E Chirwa (2011), “The Malawi agricultural input subsidy programme: 2005/06 to 2008/09.”, International Journal of Agricultural Sustainability, 9(1): 232-247.
Duflo, E, M Kremer, and J Robinson (2011), “Nudging farmers to use fertilizer: Theory and experimental evidence from Kenya”, American Economic Review, 101(6): 2350-90.
Elabed, G, and M R Carter (2018), “Ex-ante impacts of agricultural insurance: Evidence from a field experiment in Mali”, University of California at Davis.
Fabregas, R, M Kremer, J Robinson, and F Schilbach (2017), “The effectiveness of public agricultural extension: Evidence from two approaches in Kenya”, Unpublished Working Paper.
Fink, O, Q Wang, M Svensen, P Dersin, W-J Lee, and M Ducoffe (2020), “Potential, challenges and future directions for deep learning in prognostics and health management applications”, Engineering Applications of Artificial Intelligence, 92: 103678.
Foster, A D and M R Rosenzweig (2022), “Are there too many farms in the world? Labor-market transaction costs, machine capacities and optimal farm size.”, Journal of Political Economy, 130(3): 636-680.
Gharib, M H, L H Palm-Forster, T J Lybbert and K D Messer (2021), “Fear of fraud and willingness to pay for hybrid maize seed in Kenya.”, Food Policy, 102, 102040.
Gilligan, D and N Karachiwalla (2023), “Subsidies and Product Assurance: Evidence from Agricultural Technologies”, Working Paper.
Gilligan, D, N Karachiwalla and G Thai (2022), “Information, Beliefs, and Adoption: Evidence from Agricultural Inputs in Uganda”, Working Paper.
Goldstein, M, K Houngbedji, F Kondylis, M O'Sullivan, and H Selod (2018), “Formalization without certification? Experimental evidence on property rights and investment”, Journal of Development Economics, 132: 57-74.
Goldstein, M, and C Udry (2008), “The profits of power: Land rights and agricultural investment in Ghana”, Journal of Political Economy, 116(6): 981-1022.
Harou, A P, M Madajewicz, H Michelson, C A Palm, N A. Amuri, C Magomba, J M Semoka, K Tschirhart and R Weil (2022), "The joint effects of information and financing constraints on technology adoption: Evidence from a field experiment in rural Tanzania.", Journal of Development Economics, 155: 102707.
Herrman, T J, V Hoffmann, A Muiruri and C McCormick (2020), “Aflatoxin proficiency testing and control in Kenya.” Journal of Food Protection, 83(1): 142-146.
Hildebrant, N, Y Nyarko, G Romangnoli and E Soldani (2023), “Price Information, Inter-Village Networks, and “Bargaining Spillovers”: Experimental Evidence from Ghana,” Journal of Development Economics.
Hoel, J B, H Michelson, B P Norton and V Manyong (2024), “Misattribution Prevents Learning”, American Journal of Agricultural Economics, forthcoming.
Hoffmann, V and K Jones (2021), “Improving food safety on the farm: Experimental evidence from Kenya on incentives and subsidies for technology adoption”, World Development, 143: 105406.
Hoffmann, V, C M Moser, and T J Herrman (2020), “Demand for aflatoxin‐safe maize in Kenya: Dynamic response to price and advertising”, American Journal of Agricultural Economics, 103(1): 275-295.
Hoffmann, V, S Kariuki, J Pieters and M Treurniet (2018), “Can markets support smallholder adoption of a food safety technology? Aflasafe in Kenya”, International Food Policy Research Institute (IFPRI) Working Paper.
Hoffmann, V, S Kariuki, J Pieters and M Treurniet (2023), "Upside risk, consumption value, and market returns to food safety." American Journal of Agricultural Economics, 105(3): 914-939.
Horner, D, A Bouguen, M Frölich, and M Wollni (2019), “The effects of decentralized and video-based extension on the adoption of integrated soil fertility management–experimental evidence from Ethiopia” (No. w26052). National Bureau of Economic Research.
Hsu, E and A Wambugu (2023), “Can informed buyers improve goods quality? Experimental evidence from crop seeds?”, Working Paper.
J-PAL, CEGA and ATAI Policy Bulletin (2016), “Make it Rain.”, Cambridge, MA: Abdul Latif Jameel Poverty Action Lab, Center for Effective Global Action, and Agricultural Technology Adoption Initiative.
Jagnani, M, C B Barrett, Y Liu and L You (2021), “Within-season producer response to warmer temperatures: Defensive investments by Kenyan farmers”, Economic Journal, 131(633): 392-419.
Jayachandran, S, J de Laat, E F Lambin, C Y Stanton, R Audy and N E Thomas (2017), “Cash for carbon: A randomized trial of payments for ecosystem services to reduce deforestation”, Science, 357: 267-273.
Jayne, T S, J Chamberlin, S Holden, H Ghebru, J Ricker-Gilbert and F Place (2021), "Rising land commodification in sub-Saharan Africa: Reconciling the diverse narratives." Global Food Security, 30: 100565.
Jayne, T S, J Chamberlin, L Traub, N Sitko, M Muyanga, F K Yeboah, W Anseeuw (2016), "Africa's changing farm size distribution patterns: the rise of medium‐scale farms.", Agricultural Economics, 47(S1): 197-214.
Jayne, T S, N Mason, W J Burke and J Ariga (2018), “Taking stock of Africa’s second-generation agricultural input subsidy programs”, Food Policy, 75: 1-14.
Jayne, T S, S Zingore, A I Niang, C Palm and P Sanchez (2023), “Building twenty-first century agricultural research and extension capacity in Africa.” European Review of Agricultural Economics, 50(5): 1824-1846.
Jones, M, F Kondylis, J Loeser, and J Magruder (2022), “Factor market failures and the adoption of irrigation in Rwanda”, American Economic Review, 112(7): 2316-52.
Kadjo, D, J Ricker-Gilbert and C Alexander (2016), “Estimating price discounts for low-quality maize in sub-Saharan Africa: evidence from Benin.” World Development, 77: 115-128.
Kala, N (2017), “Learning, adaptation, and climate uncertainty: Evidence from Indian agriculture”, MIT Center for Energy and Environmental Policy Research Working Paper 23.
Karlan, D, E Kutsoati, M McMillan, and C Udry (2011), “Crop price indemnified loans for farmers: A pilot experiment in rural Ghana”, Journal of Risk and Insurance, 78(1): 37-55.
Karlan, D, R Osei, I Osei-Akoto, and C Udry (2014), “Agricultural decisions after relaxing credit and risk constraints”, Quarterly Journal of Economics, 129(2): 597-652.
Kondylis, F, V Mueller and J Zhu (2017), "Seeing is believing? Evidence from an extension network experiment.", Journal of Development Economics, 125: 1-20.
Krah, K, A Maertens, H Michelson, W Mhango and V Nourani (2024), “Village Fairness Norms and Land Rental Markets.”, World Bank Economic Review, forthcoming.
Kramer, B (2023), "Impacts of tailored plot-level agricultural extension and crop insurance services based on pictures." AEA RCT Registry. June 23. https://doi.org/10.1257/rct.11423-1.0
Laajaj, R, and K Macours (2021), "Measuring skills in developing countries." Journal of Human Resources, 56(4): 1254-1295.
Le Cotty, T, E M d'Hotel, R Soubeyran and J Subervie (2019), "Inventory credit as a commitment device to save grain until the hunger season.", American Journal of Agricultural Economics, 101(4): 1115-1139.
Lobell, D B, G Azzari, M Burke, S Gourlay, Z Jin, T Kilic and S Murray (2020), "Eyes in the Sky, Boots on the Ground: Assessing Satellite‐and Ground‐Based Approaches to Crop Yield Measurement and Analysis.", American Journal of Agricultural Economics, 102(1): 202-219.
Macchiavello, R, and A Morjaria (2021), “Competition and relational contracts in the Rwanda coffee chain”, Quarterly Journal of Economics, 136(2): 1089-1143.
Macchiavello, R and J Miquel-Florensa (2019), “Buyer-Driven Upgrading in GVCS: The Sustainable Quality Program in Colombia”, CEPR Discussion Paper No. DP13935.
Maertens, A, H Michelson and V Nourani (2021), “How do farmers learn from extension services? Evidence from Malawi.”, American Journal of Agricultural Economics, 103(2): 569-595.
Maertens, A, C Magomba and H Michelson (2022), “Correcting False Beliefs: Fertilizer in Tanzania”. Draft.
Magnan, N, V Hoffmann, N Opoku, G G Garrido, and D A Kanyam (2021), “Information, technology, and market rewards: Incentivizing aflatoxin control in Ghana”, Journal of Development Economics, 151: 102620.
Magruder, J (2018), “An Assessment of Experimental Evidence on Agricultural Technology Adoption in Developing Countries”, Annual Review of Resource Economics.
Matsumoto, T, T Yamano, and D Sserunkuuma (2013), “Technology adoption and dissemination in agriculture: evidence from sequential intervention in maize production in Uganda”, National Graduate Institute for Policy Studies, No. 13-14.
Michelson, H, A Fairbairn, B Ellison, A Maertens and V Manyong (2021), "Misperceived Quality: Fertilizer in Tanzania.", Journal of Development Economics, 148: 102579.
Michelson, H, C Magomba and A Maertens (2024), “Restoring Trust: Evidence from the Fertiliser Market in Tanzania.” Working Paper.
Miehe, C, R Sparrow, D Spielman and B Van Campenhout (2023), “The (perceived) quality of agricultural technology and its adoption: Experimental evidence from Uganda.”, IFPRI Discussion Paper 2198.
Mukherjee, S, L F Bergquist, M Burke and E Miguel (2022), “Unlocking the Benefit of Credit through Savings.”, Working Paper.
Nakano, Y, and E F Magezi (2020), “The impact of microcredit on agricultural technology adoption and productivity: Evidence from randomized control trial in Tanzania”, World Development, 133: 104997.
Nakasone E, M Torero and B Minten (2014), “The Power of Information: The ICT Revolution in Agricultural Development”, Annual Review of Resource Economics, 6(1): 533-550.
Newman, N, L F Bergquist, N Immorlica, K Leyton-Brown, B Lucier, C McIntosh, J Quinn and R Ssekibuule (2018), "Designing and Evolving an Electronic Agricultural Marketplace in Uganda." In COMPASS ’18: ACM SIGCAS Conference on Computing and Sustainable Societies (COMPASS).
Nyarko, Y, and H S Pellegrina (2022), “From bilateral trade to centralized markets: A search model for commodity exchanges in Africa”, Journal of Development Economics, 157: 102867.
Oliva, P, B K Jack, S Bell, E Mettetal, and C Severen (2020), “Technology adoption under uncertainty: Take-up and subsequent investment in Zambia”, Review of Economics and Statistics, 102(3): 617-632.
Omotilewa, O J, J Ricker-Gilbert, J H Ainembabazi, and G E Shively (2018), “Does improved storage technology promote modern input use and food security? Evidence from a randomized trial in Uganda”, Journal of Development Economics, 135: 176-198.
Ortiz-Bobea, A, T R Ault, C M Carrillo, R G Chambers, and D B Lobell (2021), “Anthropogenic climate change has slowed global agricultural productivity growth”, Nature Climate Change, 11(4): 306-312.
Porteous, O (2019), "High trade costs and their consequences: an estimated dynamic model of African agricultural storage and trade.", American Economic Journal: Applied Economics, 11(4): 327-66.
Prieto, S, J Ricker-Gilbert, J Bauchet, and M Sall (2021), “Incomplete information and product quality in rural markets: Evidence from an experimental auction for maize in Senegal”, Economic Development and Cultural Change, 69(4): 1351-1377.
Restuccia, D and R Santaeulalia-Llopis (2017), “Land misallocation and productivity.”, NBER Working Paper No. 23128.
Ricker‐Gilbert, J, N M Mason, F A Darko and S T Tembo (2013), “What are the effects of input subsidy programs on maize prices? Evidence from Malawi and Zambia.”, Agricultural Economics, 44(6): 671-686.
Sanabria, J, G Dimithe and E K M Alognikou (2013), “The Quality of Fertilizer Traded in West Africa: Evidence for Stronger Control.”, IFDC Report.
Sanabria, J, J Ariga, J Fugice, and D Mose (2018a), “Fertilizer Quality Assessment in Markets of Uganda”, International Fertilizer Development Center.
Sanabria, J, J Ariga, J Fugice, and D Mose (2018b), “Fertilizer Quality Assessment in Markets of Kenya”, International Fertilizer Development Center.
Sanchez, P A (2019), Properties and Management of Soils in the Tropics, London: Cambridge University Press.
Serfilippi, E, M R Carter and C Guirkinger (2020), “Insurance Contracts when Farmers “Greatly Value” Certainty,” Journal of Economic Behavior and Organization, 180: 731-743.
Sheahan, M and C B Barrett (2017), “Ten striking facts about agricultural input use in Sub-Saharan Africa.” Food Policy, 67: 12-25.
Shin, S, N Magnan, C Mullally, and S Janzen (2022), “Demand for weather index insurance among smallholder farmers under prospect theory”, Journal of Economic Behavior and Organization, 202: 82-104.
Stevenson, J R, and P Vlek (2018), “Assessing the adoption and diffusion of natural resource management practices: Synthesis of a new set of empirical studies”, CGIAR Standing Panel on Impact Assessment Synthesis Report.
Suri, T (2011), "Selection and comparative advantage in technology adoption.", Econometrica, 79(1): 159-209.
Suri, T and C Udry (2021), “Agricultural Technology in Africa”, Journal of Economic Perspectives, 36(1): 33-56.
Tarozzi, A, J Desai and K Johnson (2015), “The Impacts of Microcredit: Evidence from Ethiopia”, American Economic Journal: Applied Economics, 7(1): 54-89.
Teravaninthorn, S and G Raballand (2009), “Transport Prices and Costs in Africa: A Review of the International Corridors.”, Washington, DC: World Bank.
Tjernström, E (2017), “Learning from others in heterogeneous environments” Unpublished Manuscript.
Van Campenhout, B, D J Spielman and E Lecoutere (2021), “Information and communication technologies to provide agricultural advice to smallholder farmers: Experimental evidence from Uganda.”, American Journal of Agricultural Economics, 103(1): 317-337.
Wiseman, E (2023), “Border Trade and Information Frictions: Evidence from Informal Traders in Kenya” Working Paper.
Wren-Lewis, L, L Becerra-Valbuena, and K Houngbedji (2020), “Formalizing land rights can reduce forest loss: Experimental evidence from Benin”, Science Advances, 6(26): eabb6914.
Contact VoxDev
If you have questions, feedback, or would like more information about this article, please feel free to reach out to the VoxDev team. We’re here to help with any inquiries and to provide further insights on our research and content.