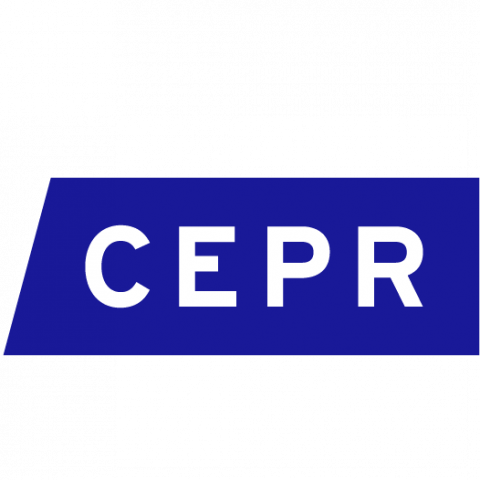
An immediate impact of new or improved transportation networks is the time saving and/or reduced costs for those using the network, and these impacts were the focus of the traditional approach in economics for evaluating the benefits of transportation investments. In a seminal paper, Fogel (1964) developed a new methodology to evaluate the historical effects of railroads on economic outcomes. In his study, he compares the cost savings from railroads relative to other transportation modes, such as water transport or wagons over land. In a similar approach, McFadden (1974) develops a cost-saving formula to evaluate the benefits of transport infrastructure by calculating average time savings weighted by the value of jobs. While these first approaches incorporate an important benefit of transportation — the implied cost and time savings — into their analysis, the more recent literature on the impacts of infrastructure has focused on confronting two central issues for the identification of causal effects: non-random placement and general equilibrium effects (Redding and Turner 2015).
Infrastructure investments are commonly allocated to places that are more desirable due to either a fundamentally higher productivity for firms or a higher amenity value for consumers. This “non-random” or “endogenous” placement of infrastructure makes it difficult for researchers to disentangle whether infrastructure causes high productivity, or if infrastructure is strategically placed where productivity is already higher or expected to grow. This implies that the use of observational data to estimate impacts will fail to capture the causal effect of investments, because it will be biased by the selection of more productive or attractive places for infrastructure.[1] Instrumental variables are one common approach which has emerged to address the challenge of endogenous placement and obtain causal estimates of the impacts of infrastructure placement.[2]
With this approach, two families of instrumental variables have emerged as predictors of transportation infrastructure placement. The first is historical route instruments (Martincus et al. 2017, Banerjee et al. 2020). The idea behind this approach is that historical roads or railroad routes can predict current infrastructure levels (relevance), and because they are a function of historical determinants, they are not a function of more recent shocks to productivity or amenities conditional on other geographic factors (exogeneity).
The other popular approach using instrumental variables relies on incidental connections. This strategy argues that the objective of large infrastructure projects is primarily to connect major points of interest — for example two large cities or a large city to a port. In the process of building such a highway or railroad, villages or cities along the way will also be connected, but for these the infrastructure is provided as if by chance (exogenously). Two variants of instruments relying on incidental connections use least cost paths (Faber 2014, Morten and Oliviera 2023, Fenske et al. 2023) or shortest routes (Banerjee et al. 2020, Jedwab and Moradi 2016, Ghani et al. 2016, Garcia-Lopez et al. 2015), as instruments for being connected. Figure 2 provides examples of straight-line and least-cost instruments from Banerjee et al. (2020) and Faber (2014) applied to study highways in the Chinese context. The straight lines drawn in Banerjee et al. (2020) are correlated with the actual road network, but are free of political or strategic placement of the true route. In Panel (b), the least-cost instrument in Faber (2014) again approximates the true network, but draws the route that would be used to minimise construction costs and should therefore be independent of strategic placement along the way.
Figure 2: Examples of straight-line and least-cost instruments applied to China
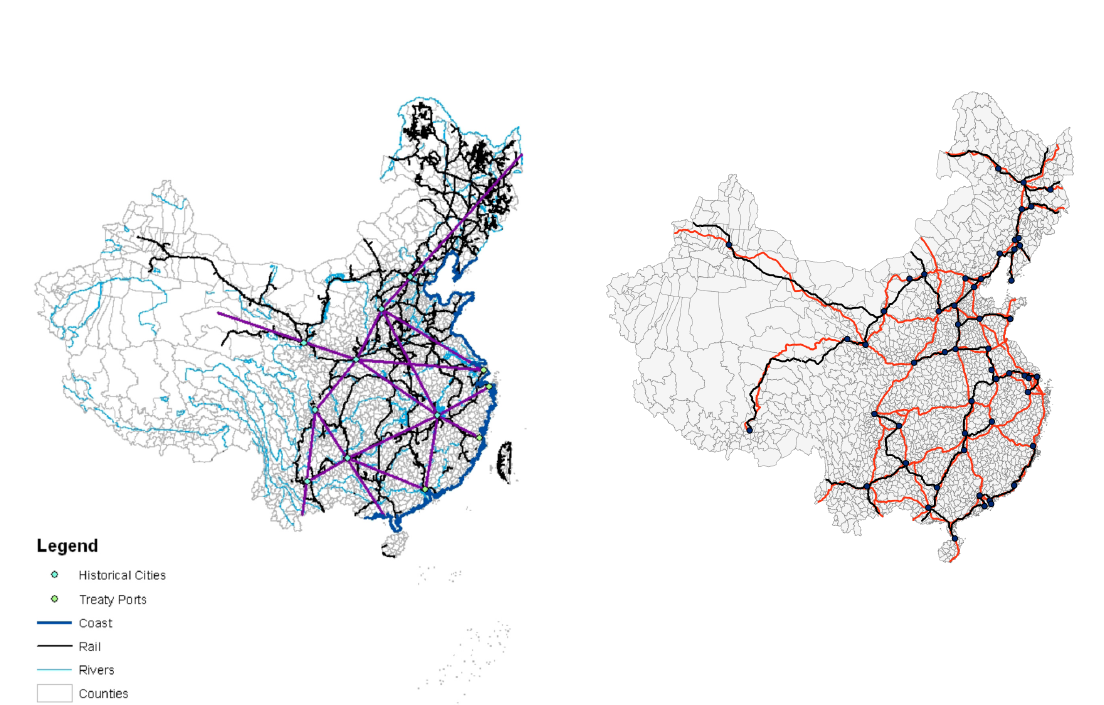
These approaches are attractive because they solve the endogenous placement problem. However, they are unable to distinguish between reallocation and growth effects, since part of the difference in outcomes caused by infrastructure could result from diverting activity away from “untreated” to “treated” areas. One empirical strategy to partially address this concern is to assume that such spillover effects are local in nature. Then we can think of two kinds of comparisons: outcomes in areas receiving infrastructure vs nearby areas (which may be affected by reallocation effects), and areas receiving infrastructure vs distant areas (which are not subject to reallocation effects). If these comparisons provide similar estimates then reallocation effects are likely weak. While these tests are informative about local reallocation effects, this strategy does not deal well with reallocation that is not local in nature, such as when a multinational corporation chooses where to locate a plant amongst multiple distant sites within a country.[3]
The other challenge that has been confronted by recent advances in the literature refers to better capturing general equilibrium effects. The development of land transportation networks can create large changes in production, prices, commuting, and other outcomes, which implies that the direct effects on time or cost savings are only part of the total gains from these investments. Recent papers have addressed this challenge by building on general equilibrium economic geography models of trade and iceberg transport costs (Allen and Arkolakis 2014, Redding and Rossi-Hansberg 2017, Allen et al. 2020, Allen and Arkolakis 2022). These models incorporate multiple regions and (costly) trade between them, making them well suited to account for indirect effects and to capture overall growth effects, as they consider impacts on both directly and indirectly exposed regions. They also help empirical approaches to aggregate direct and indirect effects, by using models to estimate “market access” measures that capture a location’s “access” to trade partners (Donaldson and Hornbeck 2016, Ahlfeldt et al. 2015).[4]
A key advantage of these models is that they explicitly incorporate indirect effects or spillovers, and provide a systematic way for thinking about how these impacts interact with market distortions. Distortions and market failures play a significant role in developing countries, and the resource misallocation that they cause means that observed allocations may not be optimal. In this kind of setting, infrastructure can have additional benefits or costs depending on how it interacts with these distortions, and general equilibrium models provide tools for characterising these interactions (Asturias et al. 2019, Balboni 2023, Zarate 2023) .
In sum, using general equilibrium models of trade and gravity combined with reduced form estimates and clever causal research designs is increasingly popular and has improved our understanding of the benefits of building infrastructure. These models have allowed researchers to disentangle growth effects from reallocation, and provide a structured — albeit model reliant — way to think about how spillovers behave when locations are connected by trade, migration, or commuting.
In the next sections, we summarise findings from the growing body of research on land transport infrastructure for different modes of interregional transportation and intracity transit.
References
Ahlfeldt, G, S Redding, D Sturm, and N Wolf (2015), “The Economics of Density: Evidence From the Berlin Wall.” Econometrica 83(6): 2127–2189.
Allen, T and C Arkolakis (2014), “Trade and the Topography of the Spatial Economy.” Quarterly Journal of Economics 129(3): 1085–1140.
Allen, T, C Arkolakis, and X Li (2020), “On the Equilibrium Properties of Network Models with Heterogeneous Agents.” NBER Working Paper 27837.
Allen, T and C Arkolakis (2022), “The Welfare Effects of Transportation Infrastructure Improvements.” Review of Economic Studies 89(6): 2911–2957.
Asher, S and P Novosad (2020), “Rural Roads and Local Economic Development.” American Economic Review 110(3): 797–823.
Asturias, J, M García-Santana, and R Ramos (2019), “Competition and the Welfare Gains from Transportation Infrastructure: Evidence from the Golden Quadrilateral of India.” Journal of the European Economic Association 17(6): 1881–1940.
Balboni, C (2023), “In Harm’s Way? Infrastructure Investments and the Persistence of Coastal Cities.” Working Paper.
Banerjee, A, E Duflo, and N Qian (2020), “On the Road: Access to Transportation Infrastructure and Economic Growth in China.” Journal of Development Economics 145: 102442.
Borusyak, K and P Hull (2023), “Non-Random Exposure to Exogenous Shocks.” Econometrica, forthcoming.
Casaburi, L, R Glennerster, and T Suri (2013), “Rural Roads and Intermediated Trade: Regression Discontinuity Evidence from Sierra Leone.” MIT Sloan Working Paper 5043-13.
Donaldson, D and R Hornbeck (2016), “Railroads and American Economic Growth: A ‘Market Access’ Approach.” Quarterly Journal of Economics 131(2): 799–858.
Faber, B (2014), “Trade Integration, Market Size, and Industrialization: Evidence from China’s National Trunk Highway System.” Review of Economic Studies 81(3): 1046–1070.
Fenske, J, N Kala, and J Wei (2023), “Railways and Cities in India.” Journal of Development Economics 161.
García-López, M, A Holl, and E Viladecans-Marsal (2015), “Suburbanization and Highways in Spain when the Romans and the Bourbons Still Shape its Cities.” Journal of Urban Economics 85: 52–67.
Ghani, E, A Grover Goswami, and W Kerr (2016), “Highway to Success: The Impact of the Golden Quadrilateral Project for the Location and Performance of Indian Manufacturing.” Economic Journal 126(591): 317–357.
Gonzalez-Navarro, M and C Quintana-Domeque (2016), “Paving Streets for the Poor: Experimental Analysis of Infrastructure Effects.” Review of Economics and Statistics 98(2): 254–267.
Jedwab, R and A Moradi (2016), “The Permanent Effects of Transportation Revolutions in Poor Countries: Evidence from Africa.” Review of Economics and Statistics 98: 268–284.
Martincus, C, J Carballo, and A Cusolito (2017), “Roads, Exports and Employment: Evidence from a Developing Country.” Journal of Development Economics 125: 21–39.
McFadden, D (1974), Conditional Logit Analysis of Qualitative Choice Behavior. In P. Zarembka (Ed.), Frontiers in Econometrics, pp. 105–142. New York: Academic Press.
Morten, M and J Oliviera (2023), “The Effects of Roads on Trade and Migration: Evidence from a Planned Capital City.” Working Paper.
Redding, S and E Rossi-Hansberg (2017), “Quantitative Spatial Economics.” Annual Review of Economics 9(1): 21–58.
Zarate, R (2023), “Spatial Misallocation, Informality, and Transit Improvements: Evidence from Mexico City.” World Bank Policy Research Working Paper 9990.
Contact VoxDev
If you have questions, feedback, or would like more information about this article, please feel free to reach out to the VoxDev team. We’re here to help with any inquiries and to provide further insights on our research and content.